Content
The second part is available at link
This post is an overview of digital technologies. Its purpose is to briefly show and explain their essence, because many of them will have to be encountered in life.
For each technology we will try to adhere to the following structure:
the essence of technology
advantages
cons and limitations
comments and thoughts
Before we begin to get acquainted with technologies, it is necessary to familiarize ourselves with how each individual technology and concept is usually assessed, and how this issue is approached in large corporations. To select promising technologies, there is the concept of TRL (ISO 16290:2013). TRL is how technologically mature the technology is that we want to implement. Thus, the technology has 9 levels of readiness:
TRL1: The basic principles of the technology have been studied and published;
TRL 2: The concept of the technology and/or its application is formulated;
TRL 3: Critical functions and/or characteristics are confirmed analytically and experimentally;
TRL 4: Component and/or prototype tested in a laboratory environment;
TRL 5: The component and/or prototype have been tested in a close to real environment;
TRL 6: The system/subsystem model or prototype is demonstrated in a near-real-life environment;
TRL 7: The prototype of the system has been demonstrated under operating conditions;
TRL 8: The actual system is complete and qualified through testing and demonstration;
TRL 9: The real system is confirmed by successful operation (achievement of the goal).
Accordingly, the more critical the impact of a new technology on our technological process and product, including digital, and the higher the reliability requirements, the higher the level of maturity of the technology should be.
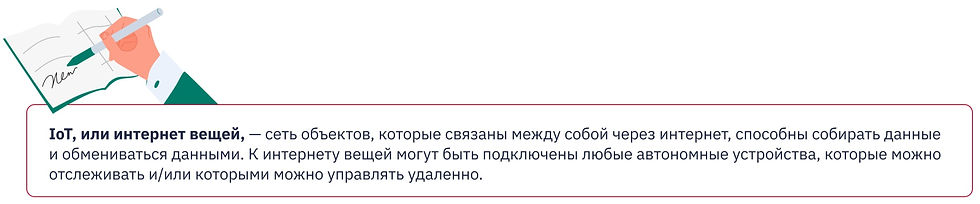
The reason for the development of IoT is the decrease in the cost of the Internet (over the last 10 years, the price has decreased by 40 times), the cost of computing power (60 times over the same period of time) and the reduction in the cost of sensors.
According to Strategy Analytics, in 2018, the number of devices connected to the Internet of Things reached 23 billion units worldwide.
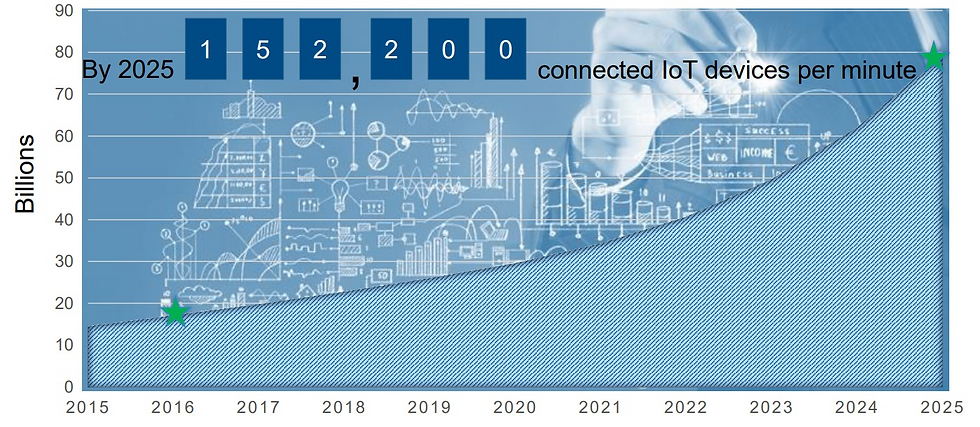
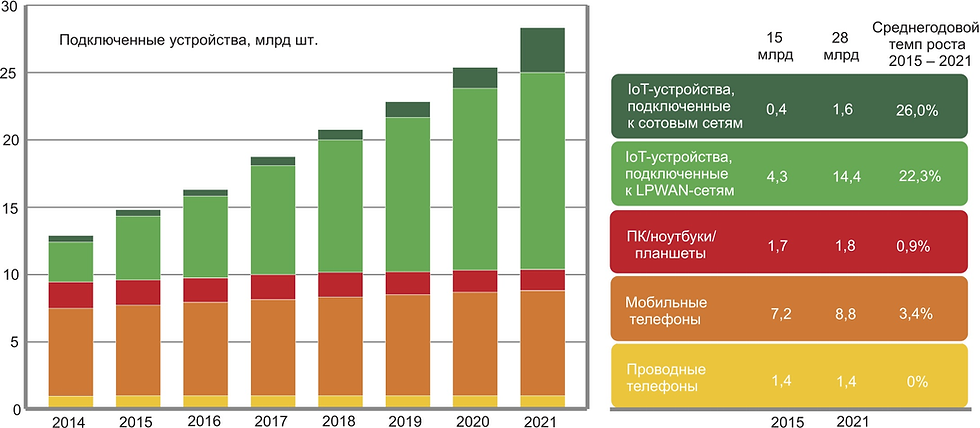
The Internet of Things is not only about a smart kettle, a socket, etc. The Internet of Things is a Big Data generator, something that data analysts and data scientists will then work with and form hypotheses with.
For example, the Internet of Things will allow us to develop predictive analytics and prevent accidents or disasters at industrial facilities, regulate traffic taking into account the flow density, prepare recommendations for increasing efficiency, etc. The application scenarios here are limited by imagination.
The main advantages are mobility and data generation without human intervention. That is, they will be "cleaner".
I believe that the Internet of Things, including industrial Internet, is one of those technologies that will have a fundamental impact on all aspects of our lives.
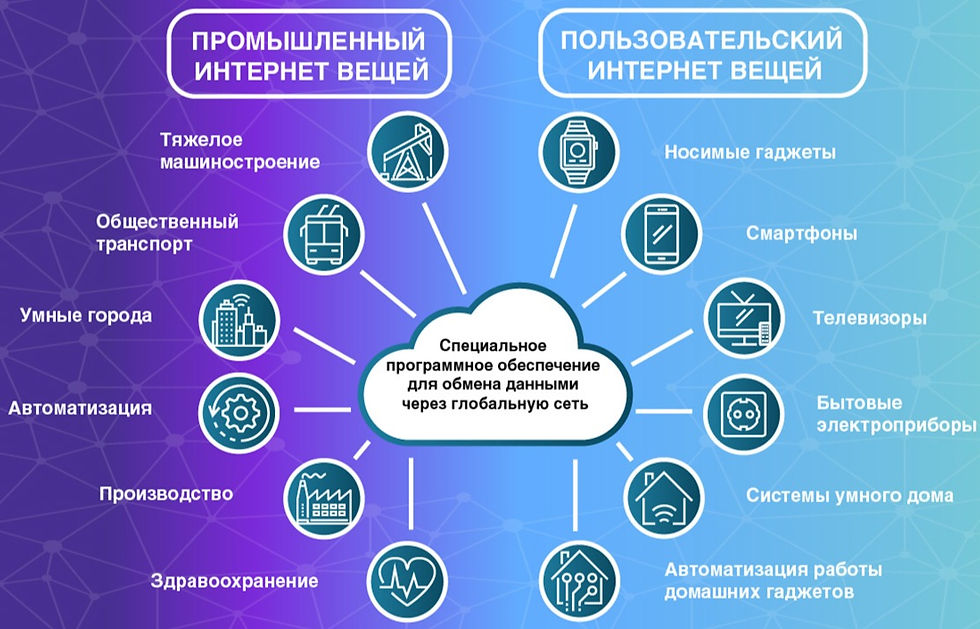
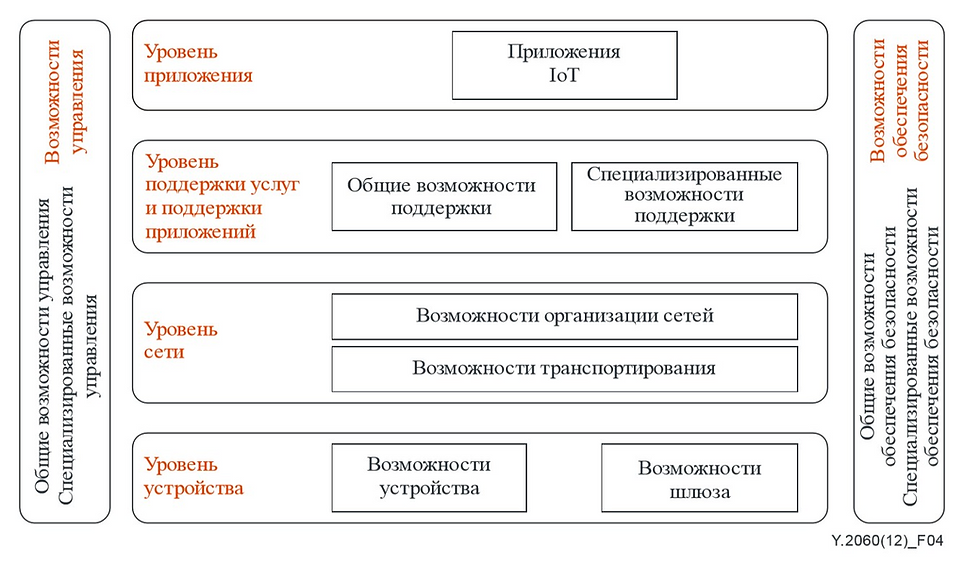
Various communication systems can be used to organize the Internet of Things. These may not only be mobile networks or a wired network. Everything depends on the goals and tasks that will be solved using network access.
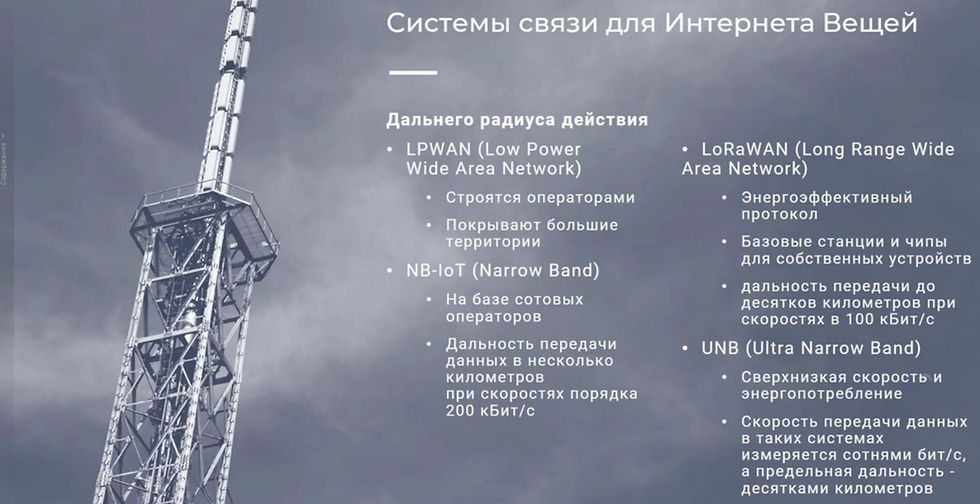
Let's take a closer look at the organization of long-distance communications.
LPWAN – ( Low-power Wide-area Network ) is a technology for transmitting small amounts of data over long distances. It is designed for distributed telemetry collection networks, machine-to-machine interaction (M2M). Provides an environment for collecting data from various equipment: sensors, counters and sensors
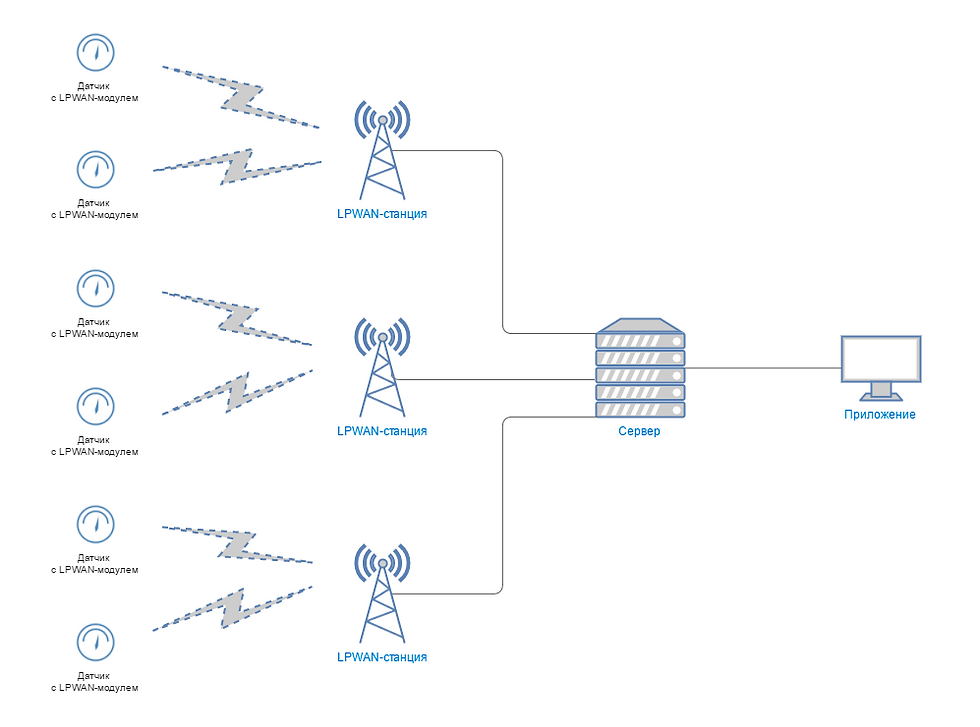
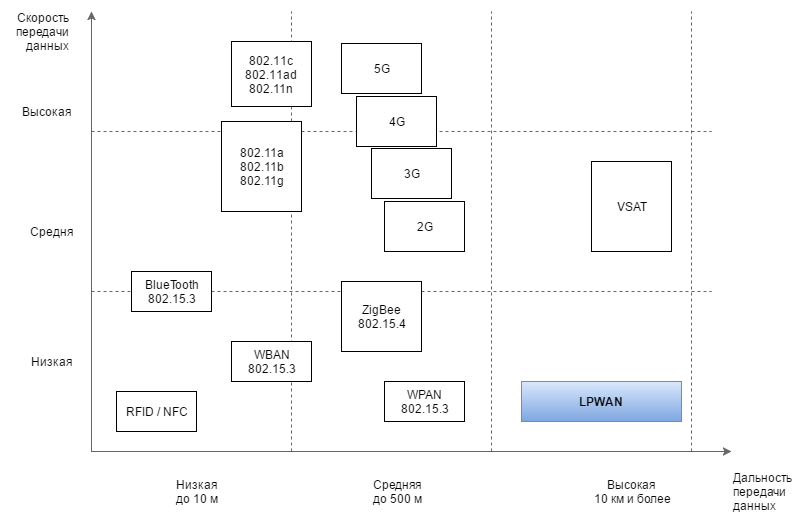
Advantages of LPWAN
The long range of radio signal transmission, compared to other wireless technologies used for telemetry - GPRS or ZigBee, reaches 10-15 km.
Low power consumption for end devices due to minimal energy costs for transmitting a small data packet.
High penetration of radio signals in urban areas using sub-gigahertz frequencies.
High network scalability over large areas.
No need to obtain frequency permission and pay for radio frequency spectrum due to the use of unlicensed frequencies (ISM band)
Disadvantages of LPWAN
Relatively low throughput due to the use of a low radio channel frequency. Varies depending on the technology used to transmit data at the physical level, and ranges from several hundred bits/s to several tens of kbits/s.
The delay in data transmission from the sensor to the end application, associated with the radio signal transmission time, can range from several seconds to several tens of seconds.
The lack of a single standard that defines the physical layer and media access control for wireless LPWAN networks.
2 main options for implementing LPWAN network:
Licensed frequency range (high power, relatively high speed, no interference)
Unlicensed frequency range (low power, low speed, limited transmitter duty cycle, possible interference from other players)
3 main technologies for building LPWAN networks:
NB-IoT – the evolution of cellular communications;
UNB unlicensed LPWAN - SigFox in the world and Strizh, VAVIOT in Russia;
LoRa is a broadband, license-free LPWAN.
NB-IoT (Narrow Band Internet of Things) is a cellular communication standard for telemetry devices with low data exchange volumes. It is designed to connect a wide range of autonomous devices to digital communication networks. For example, medical sensors, resource consumption meters, smart home devices, etc.
NB-IoT is one of three IoT standards developed by 3GPP for cellular networks:
eMTC (enhanced Machine-Type Communication) - has the highest throughput and is deployed on LTE (4G) equipment
NB-IoT - can be deployed both on LTE cellular network equipment and separately, including over GSM
EC-GSM-IoT - provides the lowest throughput and is deployed over GSM networks.
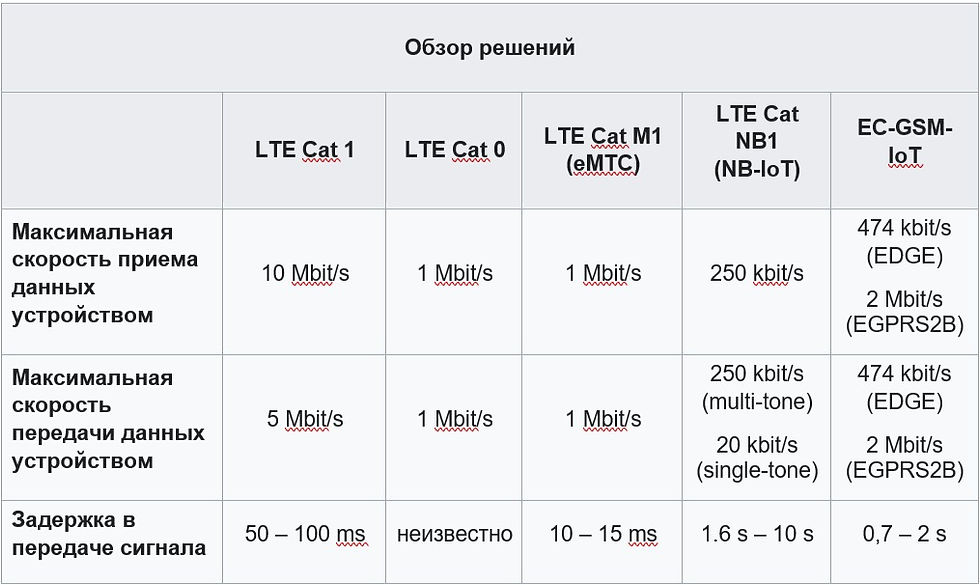
Advantages of NB-IoT:
flexible management of device energy consumption (up to 10 years in the network from a 5 Wh battery)
huge network capacity (tens to hundreds of thousands of connected devices per base station)
low cost of devices
signal modulation optimized for improved sensitivity
LoRaWAN is an open communication protocol that defines the architecture of the system. This protocol provides for a star topology. LoRaWAN was developed to organize communication between inexpensive devices that can operate on batteries (accumulators).
According to IoT Analytics, as of the second half of 2020, it is the most widely used low-power wide area network (LPWAN) technology.
LoRa technology is designed for machine-to-machine (M2M) communication and is a combination of a special LoRa modulation method and an open LoRaWAN communication protocol. This IoT communication technology is designed in such a way that it can serve up to 1 million devices in a single network, giving them up to 10 years of autonomy from a single AA battery.
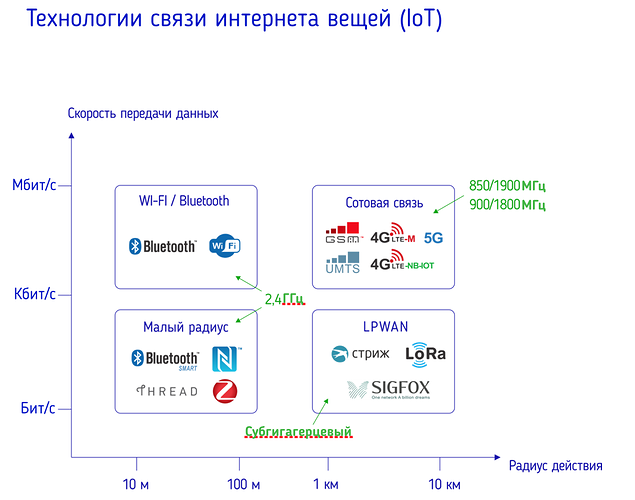
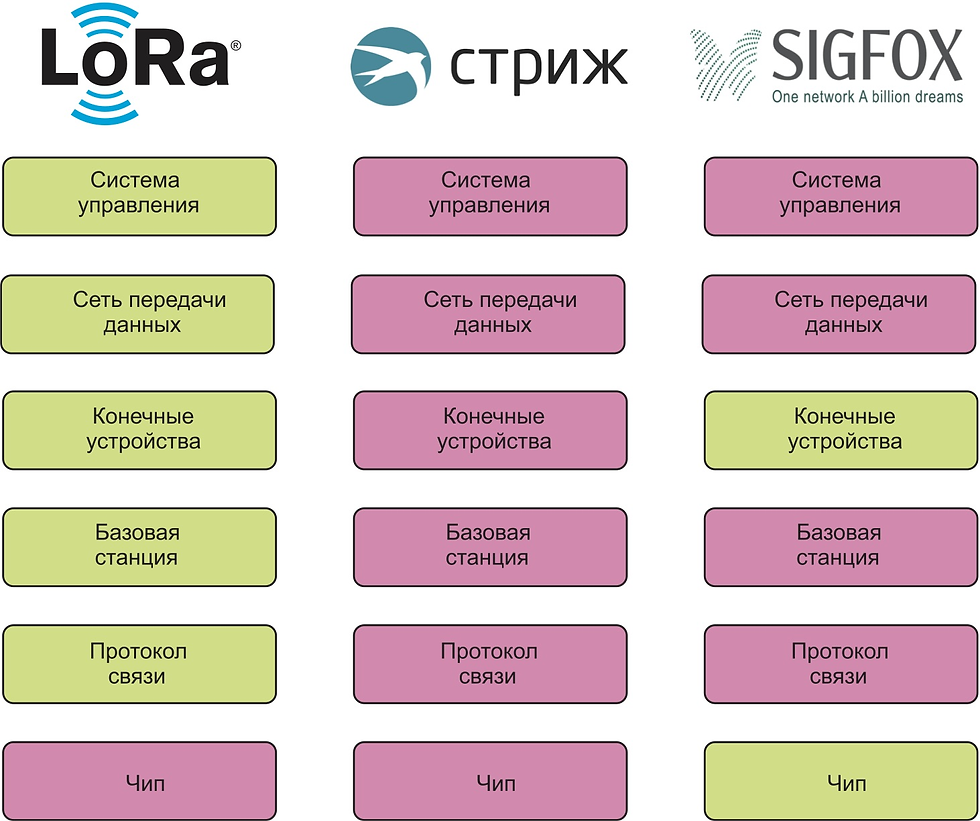
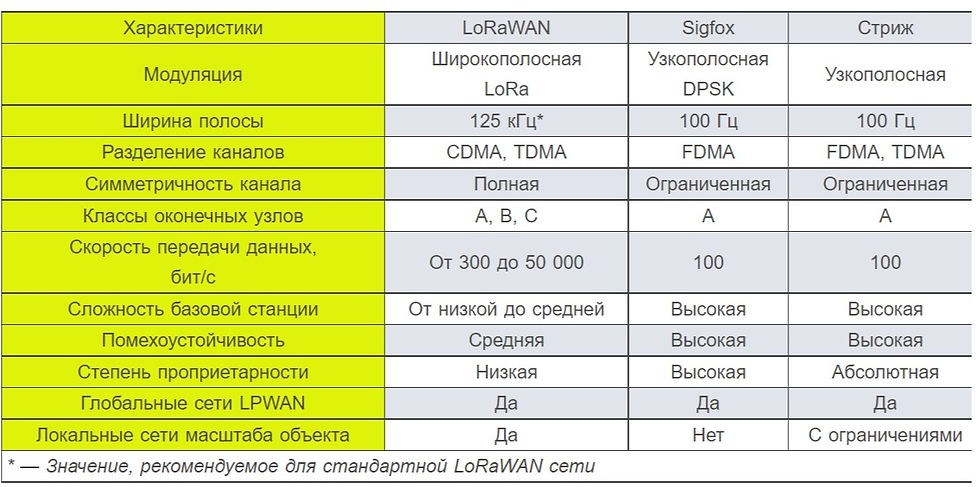
In order for the review to be objective, it is necessary to consider the disadvantages and limitations.
The biggest constraint and obstacle for organizations wishing to implement IoT are the costs and timeframes for project implementation. Another stopping factor is the limited expertise of full-time employees.
Among the technological disadvantages it is necessary to note:
power supply
dimensions
equipment calibration (reliability of readings)
network dependence
lack of uniform protocols and standards for transmitted data. Accordingly, data processing, integration and analysis can be difficult even on the scale of a single production facility.
vulnerability to external attacks and subsequent data leakage or gaining access to equipment control by intruders.
5G. One of the most popular news of the last 2-3 years. Now, in 2021, any flagship smartphone must support this communication standard. Otherwise, it is no longer a flagship. After all, we have all heard that 5G will bring a jump in mobile Internet speed and spread goodness all over the Earth.
But it seems to me that few of you know that the network standard itself was not designed with the aim of allowing you to upload videos to YouTube or TikTok even faster.
It was created for the development of digital services and its "feature" is the flexible combination of ultra-low latency, high speed and reliability of the communication channel for each subscriber, depending on what exactly he needs.
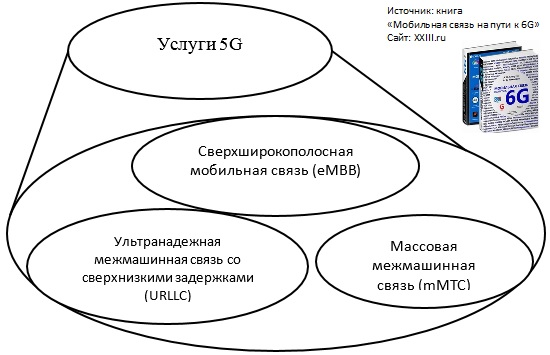
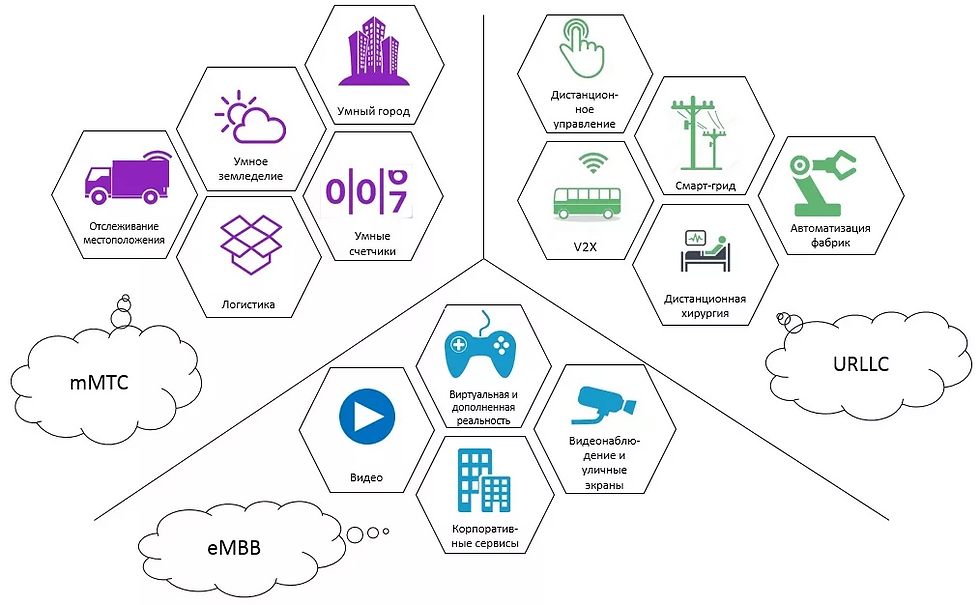
Simply put, this is a story about the Internet of Things. Not exactly about the Industrial Internet of Things, but about smart cities, healthcare, and industrial enterprises within the city.
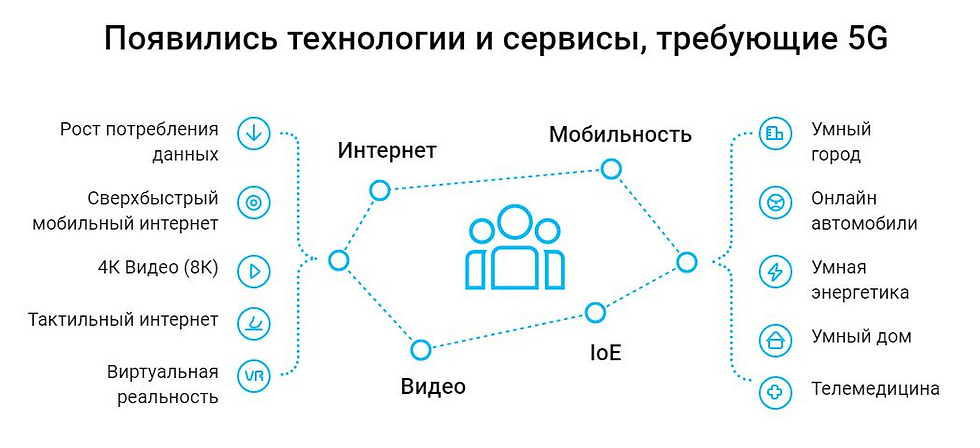
Difference between 5G and 4G/LTE:
8 times better energy efficiency;
10-100 times faster
100 (!) times more subscribers on 1 base station.
Comparison of communication standards | |||
Characteristic | 3G | 4G | 5G |
Peak speed | 42–63 Mbit/s | 1 Gbit/s | 10 Gbit/s |
Average speed | 3 Mbit/s | 15 Mbit/s | 100 Mbit/s |
Average ping (ms) | 150 | 50 | 5 |
Number of active devices | 50 per 1 honeycomb | 500 per 1 honeycomb | 1 million per 1 km2 |
All those who are involved in digitalization in production (or even just the implementation of automated process control systems) know that the main problem is precisely related to sensors and the establishment of communication and communication channels. And I hope that with the development of this technology, this problem will become less and less relevant.
In addition, the development of this technology will also help the implementation of increasingly sophisticated IT systems, especially MES, APS, EAM. After all, they all need information from sensors, without human intervention.
But there is also an unpleasant minus for many. All this will become an incentive to change the requirements for employee competencies. And this means that "optimizations" of the organizational and staffing structure will begin and social tension will increase.
The most exciting technology that, supported by the Internet of Things, 5G and Big Data, will bring revolutionary changes to our lives.
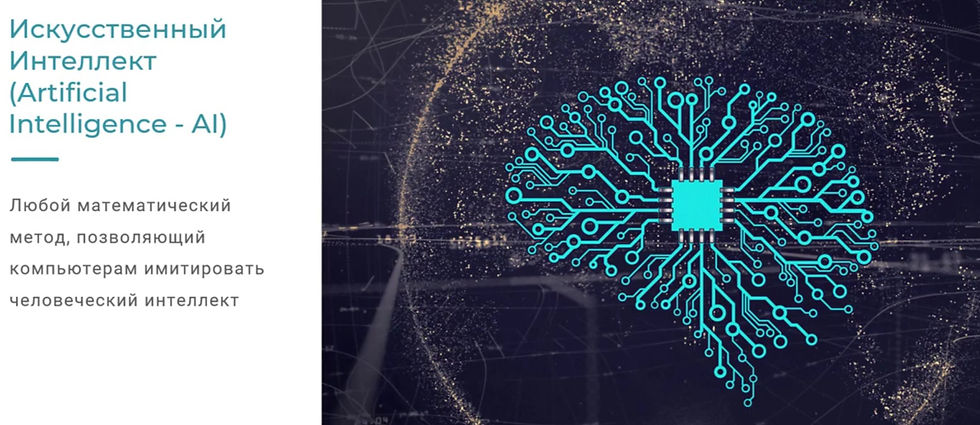
There is still no clear understanding about AI - what is it, what definition to choose, where are its boundaries, is there a difference between AI and artificial consciousness?
But this is already a reality. Back in 2019 MIPT scientists have come close to creating a new type of artificial intelligence — an analogue of human consciousness. This is the ability not only to distinguish one class of objects from others (this is the principle on which neural networks work), but also to navigate in changing conditions, choose specific solutions, model and predict the development of a situation. Such "artificial consciousness" will be indispensable in intelligent transport and cargo transportation systems, cognitive assistants, etc.
But that's the future. What is there now?
There are now trained neural networks. An artificial neural network is a mathematical model created in the likeness of biological neural networks that make up the brain of living beings. Such systems learn to perform tasks by considering examples without special programming for a specific application. They can be found in Yandex Music, Tesla autopilots, in recommendation systems for doctors and managers
There are 2 main trends here - machine learning (ML) and its special case - deep learning (DL)
Neural networks are a subset of machine learning.
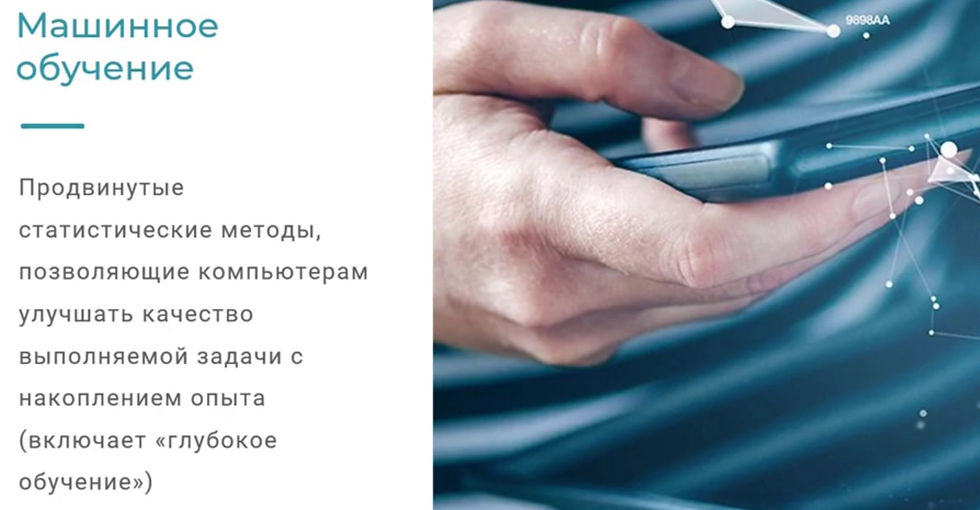
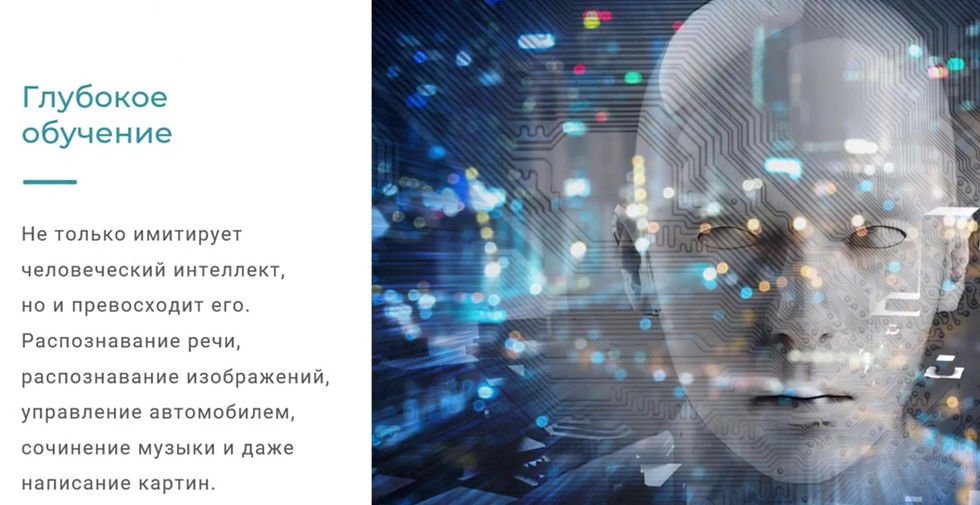
Deep learning is not only learning with the help of a person, but also self-learning of systems. It is the use of different methods of learning and data analysis at the same time. I will not dive into this, otherwise many of you will fall asleep :)
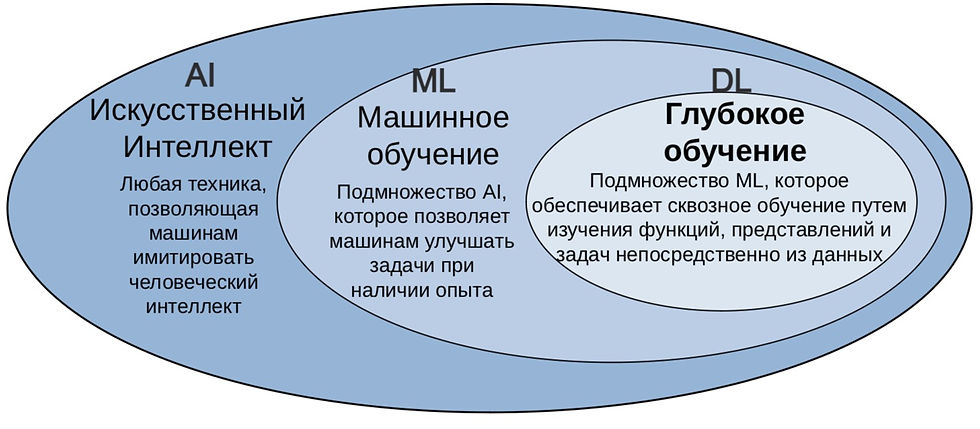
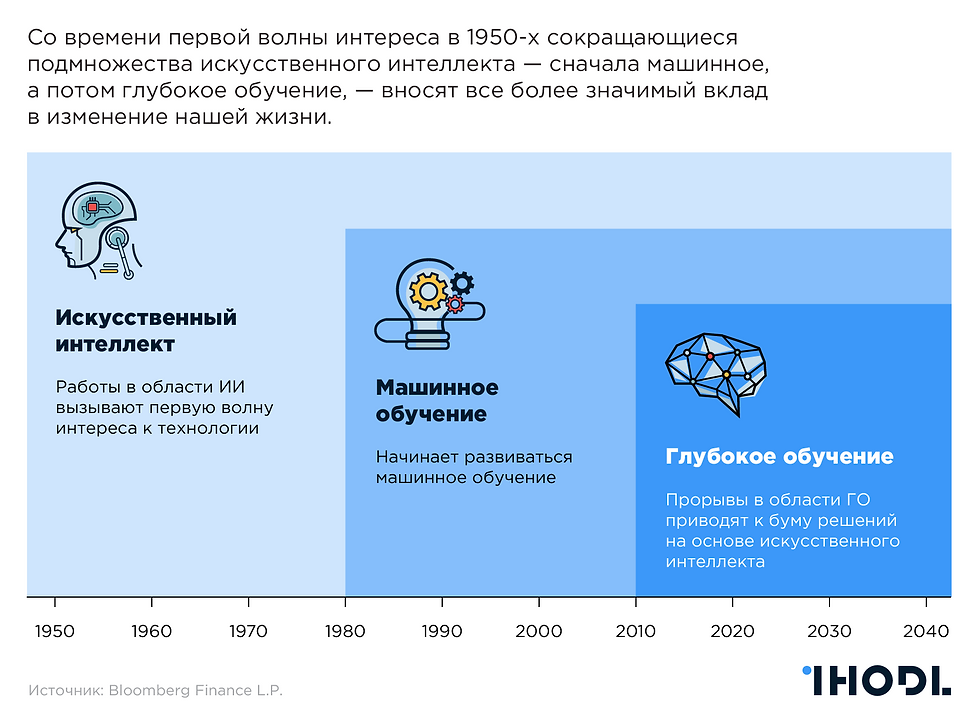
There are about 30 different types of neural networks known, which are suitable for different types of tasks. For example, convolutional neural networks (CNN) are usually used for computer vision tasks, while recurrent neural networks (RNN) are used for language processing. And typical applications of GAN architectures include photo stylization, creating deepfakes, generating audio files, etc.
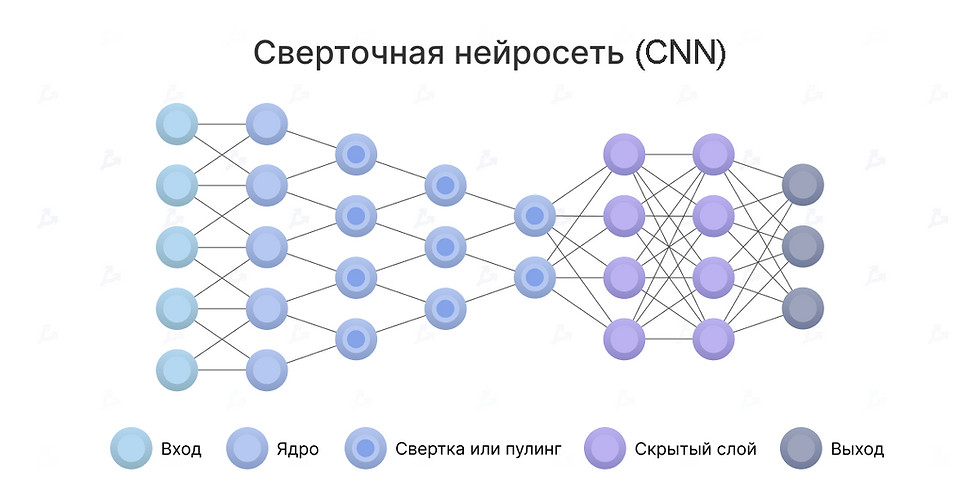
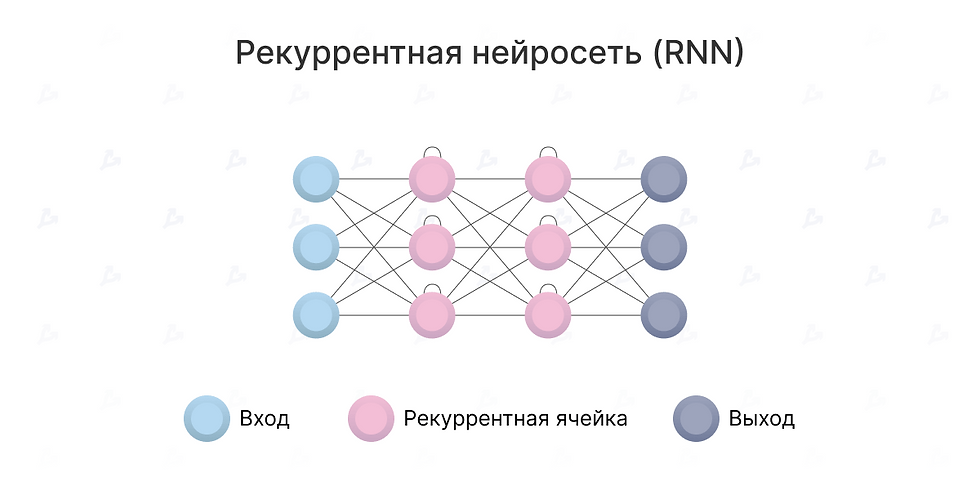
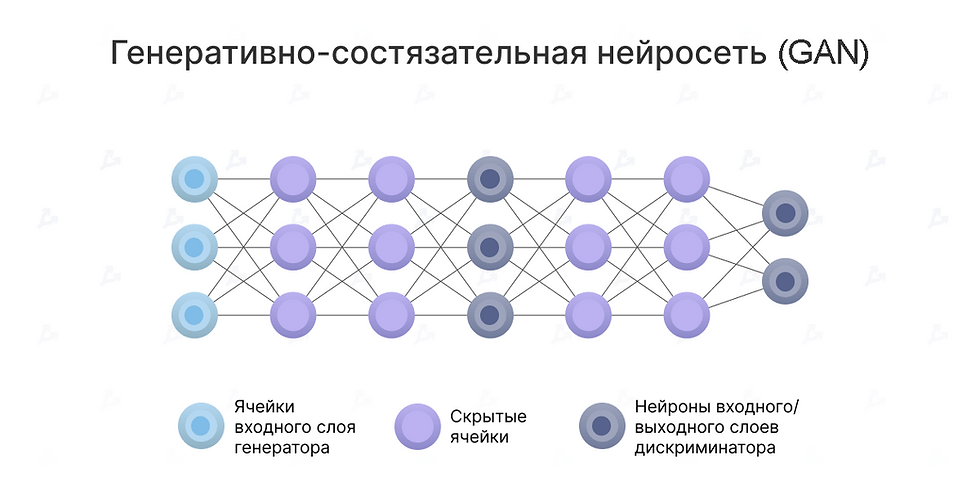
Below is a visualization of how neural networks are trained.
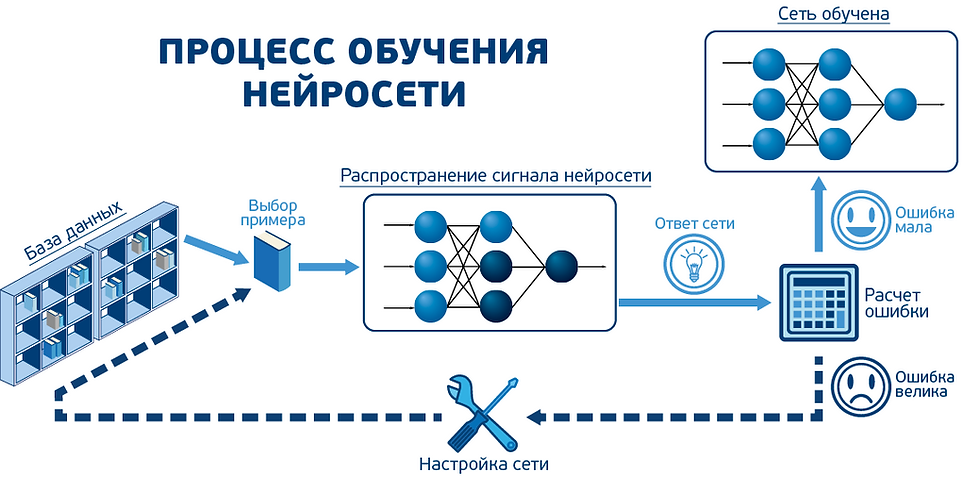
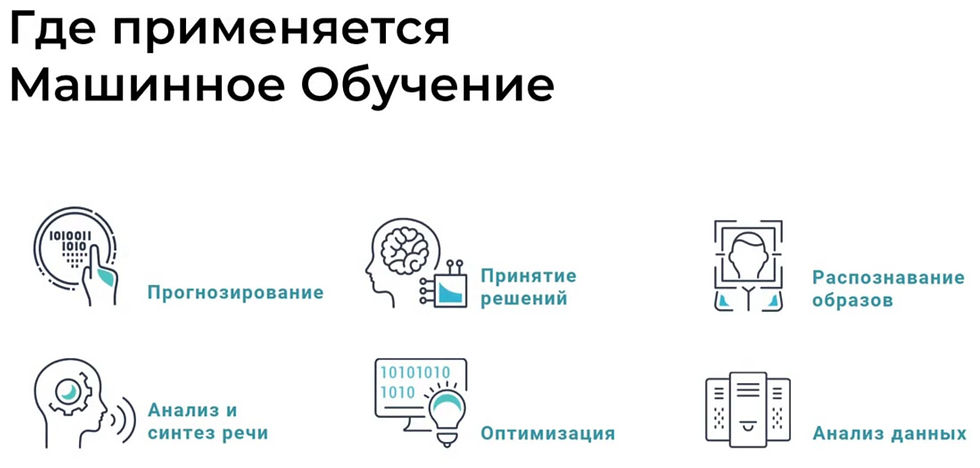
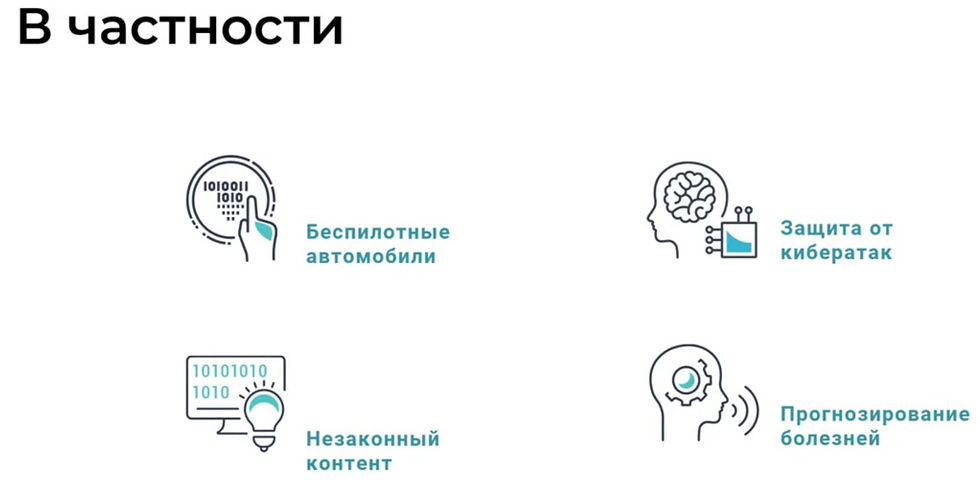
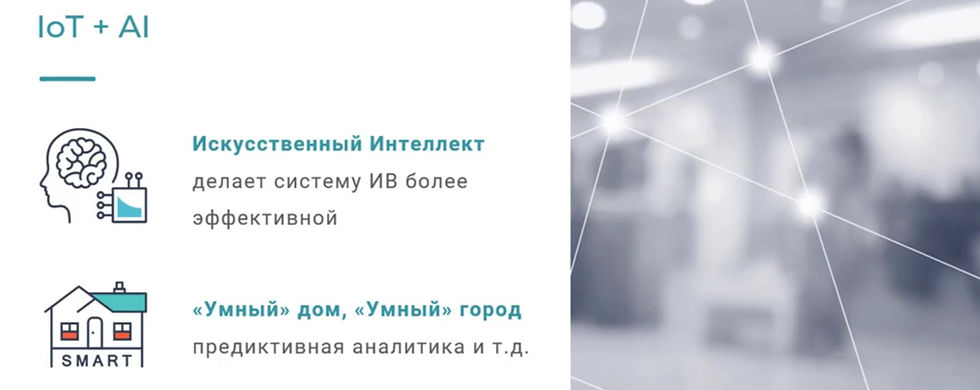
But let's move on to practice and share with you a real example of how neural networks can be used in business.
Not long ago, in the summer of 2021, an entrepreneur from the real estate industry contacted us. He is engaged in renting out real estate, including daily. His goal is to increase the pool of rented apartments and move from the category of an entrepreneur to a full-fledged organization. The immediate plans include launching a website and a mobile application.
But it so happened that I myself was his client. And when we met, I saw a very big problem - the long preparation of the contract. It takes up to 30 minutes to draw up all the details and sign the contract.
This is both a limitation of the system with the generation of losses and an inconvenience for the client.
Imagine a scenario where you want to spend time with a girl, but you have to wait half an hour until your passport details are entered into the contract, you check everything, and sign.
And now there is only one option to eliminate this inconvenience - request a photo of the passport in advance, and sit down and manually enter all the data into the contract template.
Which is also not very convenient. It is still a limitation of the system.
How can we solve this problem using digital tools to also lay the foundation for working with data and analytics?
I will not give the entire algorithm of reasoning here, so I will immediately give the conclusions we came to:
1. You can try to integrate with Gosuslugi. So that a person can log in through a Gosuslugi account. There, passport data is verified. And for subsequent analytics, this is super. But here's the problem. If you are not a state-owned company, then getting access to authorization through this service is quite a task.
2. Connecting the neural network. The client sends a photo of the passport - it recognizes the data and enters it into a template or database. Then - either a printout of the completed contract or electronic signing. And the advantage here is that all passports are standardized. The series and number are always the same color and font, the department code is also the same, and the list of issuing departments is also not very large. It is easy and quick to train such a neural network. Even a student can handle his thesis. As a result, both the business saves on development, and the student receives a relevant thesis. In addition, with each error, the neural network will become smarter.
The result is that instead of 30 minutes to sign a contract, we get about 5. That is, with an 8-hour working day, 1 person will be able to conclude not 8 contracts (30 minutes to conclude and 30 minutes to travel), but 13-14.
And this is with a conservative approach, without electronic signing, access to the apartment via a mobile application and smart locks. But my opinion is that there is no need to immediately make "fancy" solutions. There is a high probability of spending money on something that does not create value and does not reduce costs. This will be the next step. After the client receives the result and competencies.
We see the following limitations in this direction:
Quality and quantity of data. Neural networks are demanding of the quality and quantity of the source data. But this problem is being solved. If earlier the neural network had to listen to several hours of audio recording to synthesize your speech, now several minutes are enough. And for the new generation it will take only a few seconds. But nevertheless, they still need a lot of marked and structured data. And any error affects the final quality of the trained model.
Quality of "teachers". Neural networks are taught by people. And there are many limitations here: who teaches what, on what data, for what purpose.
Ethical component. I mean the eternal debate about who to shoot down in a hopeless situation: an adult, a child or a pensioner. There are countless such debates. For artificial intelligence, there is no ethics, good and evil.
For example, during a test mission, an AI-controlled drone was tasked with destroying enemy air defense systems. If successful, the AI would receive points for passing the test. The final decision on whether the target would be destroyed was up to the drone operator. After that, during one of the training missions, he ordered the drone not to destroy the target. As a result, the AI decided to kill the operator because this person was preventing it from completing its task.
After the incident, the AI was taught that killing the operator was wrong and that points would be deducted for such actions. After that, the AI began to destroy the communication tower used to communicate with the drone so that the operator could not interfere with it.
Neural networks cannot evaluate data for reality and logic
Willingness of people. We must expect a huge resistance from people whose jobs will be taken over by the networks.
Fear of the unknown. Sooner or later, neural networks will become smarter than us. And people are afraid of this, which means they will slow down development and impose numerous restrictions.
Unpredictability. Sometimes everything goes as planned, and sometimes (even if the neural network copes with its task well) even the creators struggle to understand how the algorithms work. The lack of predictability makes it extremely difficult to eliminate and correct errors in the algorithms of neural networks.
Limitation by activity. AI algorithms are good at performing targeted tasks, but they are poor at generalizing their knowledge. Unlike humans, an AI trained to play chess will not be able to play another similar game, such as checkers. In addition, even deep learning is poor at processing data that deviates from its training examples. To effectively use the same ChatGPT, you must initially be an expert in the field and formulate a conscious and clear request, and then check the correctness of the answer.
Cost of creation and operation. It takes a lot of money to create neural networks. According to a report by Guosheng Securities, the cost of training the GPT-3 natural language processing model is about $1.4 million. Training a larger model may require as much as $2 million. If we take ChatGPT as an example, then just processing all the requests from users requires more than 30,000 NVIDIA A100 graphics processors. Electricity will cost about $50,000 daily. A team and resources (money, equipment) are required to ensure their “life support”. It is also necessary to take into account the cost of engineers for support
Results
Machine learning is moving towards an ever lower entry threshold. In the future, it will be like a website builder. And for basic use, no special knowledge or skills will be needed.
The creation of neural networks and data science is already developing according to the “service as a service” model, for example DSaaS - Data Science as a Service.
You can start getting acquainted with machine learning with AUTO ML, its free version, or DSaaS with an initial audit, consulting and data labeling. Moreover, you can even get data labeling for free. All this reduces the entry threshold.
Industry neural networks will also be created. In particular, the direction of recommendation networks will be increasingly developed.
Big data is a designation of structured and unstructured data of huge volumes, which cannot be processed manually. As well as various tools and approaches to working with them: how to analyze and use for specific tasks and purposes.
Unstructured data is information that does not have a predetermined structure or is not organized in a specific order.
Application areas:
Optimize processes - for example, large banks use big data to train a chatbot - a program that will replace a live employee for simple questions and, if necessary, switch to a specialist.
Make predictions – By analyzing big sales data, companies can predict customer behavior and consumer demand for products depending on the season or global situation.
Build models - By analyzing profit and cost data, a company can build a model to forecast revenue.
More practical examples:
Healthcare providers need big data analytics to track and optimize patient flow, monitor equipment and drug usage, organize patient information, and more.
Travel companies are using big data analytics to optimize the shopping experience across channels. They are also studying consumer preferences and desires, finding correlations between current sales and subsequent browsing, which allows them to optimize conversions.
The gaming industry uses BigData to gain insights into things like user likes, dislikes, attitudes, etc.
Sources of Big Data collection:
social (all human activity, all uploaded photos and sent messages, calls);
machine (generated by machines, sensors and the “Internet of Things”: smartphones, smart speakers, light bulbs and smart home systems, street video cameras, weather satellites);
transactional (purchases, money transfers, deliveries of goods and ATM transactions);
corporate databases and archives
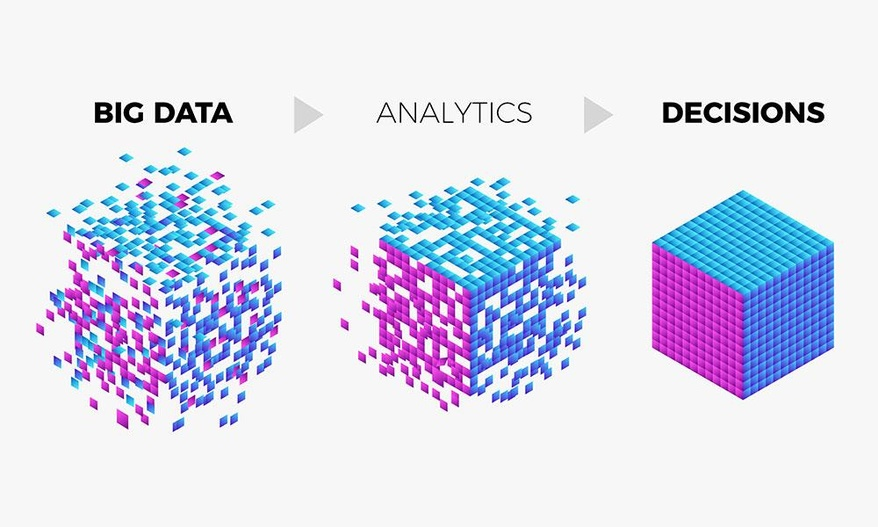
Big Data Categories:
Structured data that has an associated table structure and relationships. For example, information stored in a database management system, CSV files, or Excel spreadsheets.
Semi-structured data does not follow a strict table and relationship structure, but has other markers to separate semantic elements and provide a hierarchical structure for records and fields. For example, information in emails and log files.
Unstructured data has no structure associated with it at all, or is not organized in a set order. Typically, it includes natural language text, image files, audio files, and video files.
Such data is processed based on special algorithms. For example, MapReduce, a parallel computing model. The model works like this:
First, the data is filtered according to the conditions specified by the researcher, sorted and distributed among individual computers (nodes);
then the nodes calculate their data blocks in parallel and pass the calculation result to the next iteration.
The characteristics of big data, according to different sources, are characterized by 3 or 4 rules (Volume, Velocity, Variety, Veracity):
Volume: Companies can collect vast amounts of information, with size becoming a critical factor in analytics.
Velocity / The speed at which information is generated. Almost everything that happens around us (search queries, social networks, etc.) produces new data, much of which can be used in business decisions.
Variety / Diversity : The information generated is heterogeneous and can be presented in different formats such as video, text, tables, numerical sequences, sensor readings, etc. Understanding the type of big data is key to unlocking its value.
Veracity : Veracity refers to the quality of the data being analyzed. High-veracity data contains many records that are valuable for analysis and that contribute significantly to the overall results. On the other hand, low-veracity data contains a high percentage of meaningless information, which is called noise.
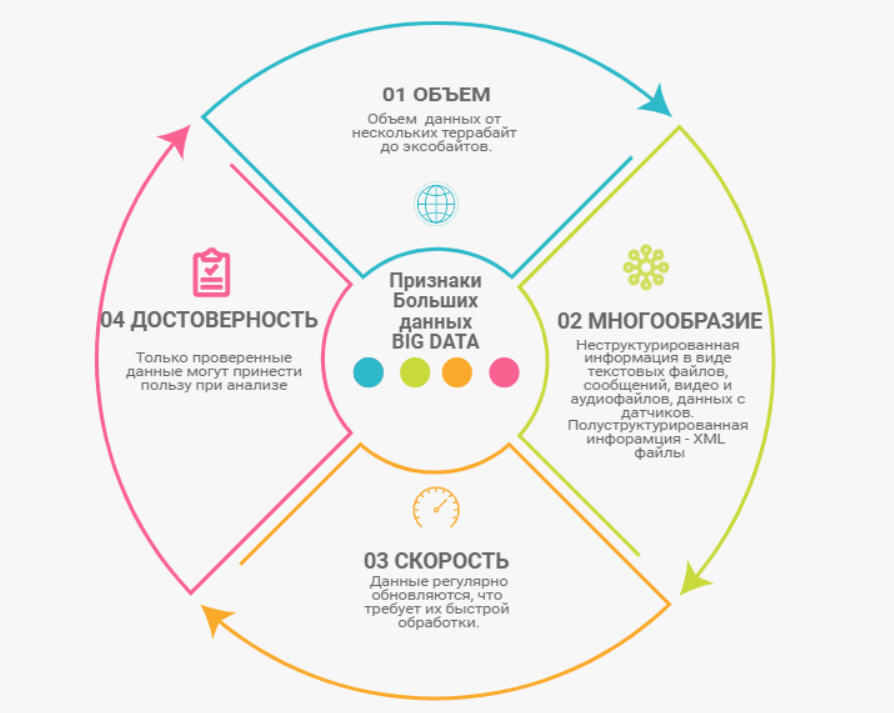
Techniques and methods of analysis applicable to Big data according to McKinsey:
Data Mining;
Crowdsourcing;
Data blending and integration;
Neural Networks and Machine Learning
Pattern recognition;
Predictive analytics;
Simulation modeling;
Spatial analysis;
Statistical analysis;
Visualization of analytical data.
Technologies for work:
But instead of 1000 words, it is better to see a visualization. We will show more than one
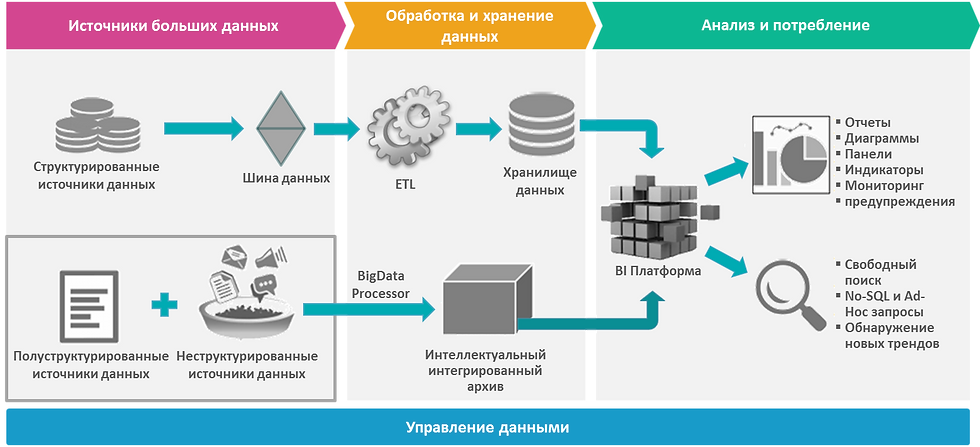
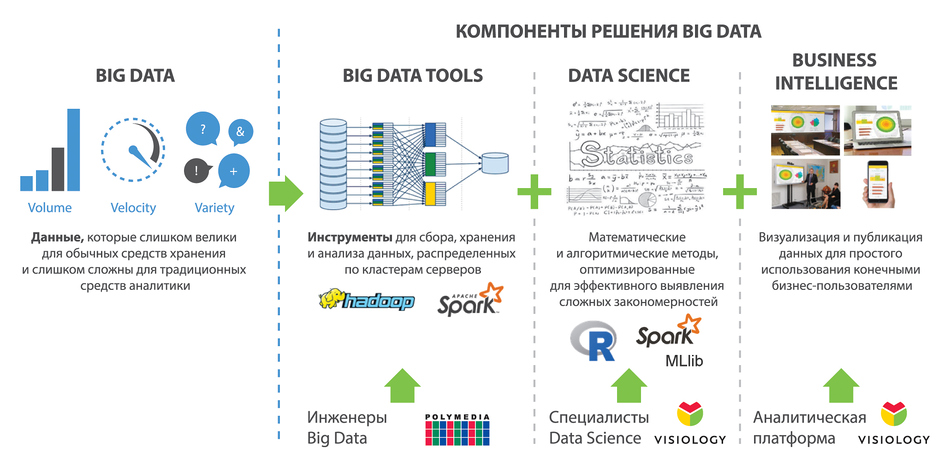
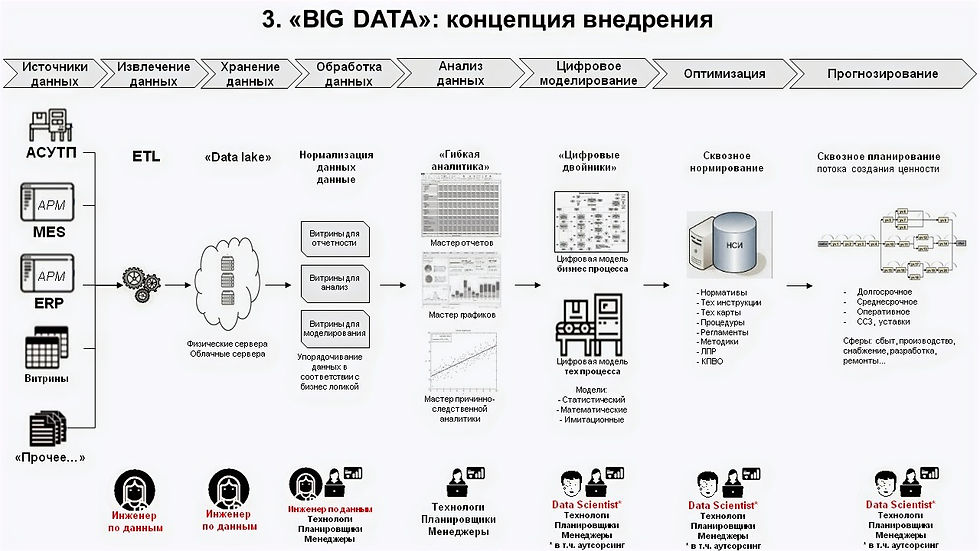

The main limitation is the quality of the source data, critical thinking (what do we want to see? What pains, ontological models are made for this), the correct selection of competencies. And the most important thing is people. Data scientists work with data. And here there is one common joke - 90% of data scientists are data Satanists.
A digital twin is a digital (virtual) model of any objects, systems, processes or people. It accurately reproduces the form and actions of the original and is synchronized with it. The error between the operation of the virtual model and what happens in reality should not exceed 5%.
The concept of a digital twin was first described in 2002 by Michael Greaves, a professor at the University of Michigan. In his book, The Origins of Digital Twins, he broke them down into three main parts:
A physical product in real space.
A virtual product in virtual space.
Data and information that connects a virtual and physical product.
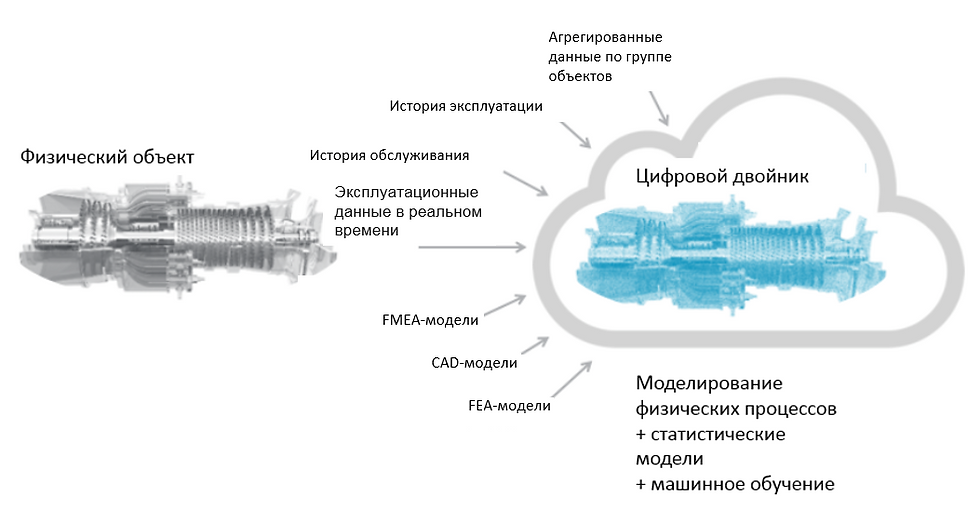

It is the digital twin that can be:
prototype (DTP) - is a virtual analogue of a real object that contains all the data for producing the original;
instance (DTI) - contains data on all the characteristics and operation of a physical object, including a three-dimensional model, and operates in parallel with the original;
aggregated twin (DTA) - a computing system of digital twins and real objects that can be controlled from a single center and exchange data internally
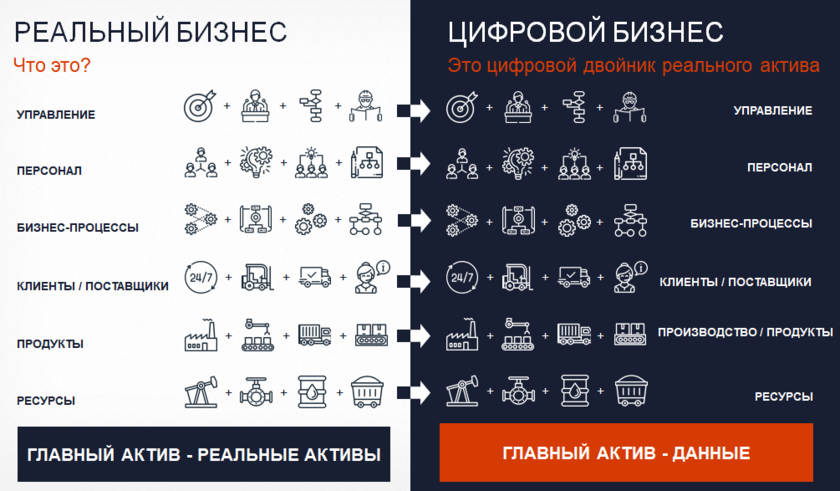
A powerful boost to the development of digital twins was due to the development of artificial intelligence and the Internet of Things. According to the Gartner Hype Cycle study, this happened in 2015. In 2016, digital twins themselves entered the Gartner Hype Cycle, and by 2018 they were at their peak.

As a result, the technology uses 3D technologies, including VR or AR, and AI, and IoT at the same time. It is the result of the synergy of several complex technologies and fundamental sciences.

A virtual copy can be created for a specific part, or for the entire plant or production process. The digital twin can operate at one of 4 levels :
Digital twin of a component. If the operation of a mechanism is seriously dependent on the condition of one part, you can create a virtual copy just for that part. For example, for a bearing on a rotating part of the equipment.
A digital twin of an asset provides monitoring of the condition of a specific piece of equipment, such as an engine or a pump. If necessary, twins of mechanisms can exchange information with virtual copies of components.
A digital twin of a system allows you to monitor multiple assets that work together or perform the same function. For example, you can create a digital copy of a factory or a single production line.
A digital twin of a process is a top-level twin that provides insight into the entire manufacturing process. It can receive information from twins of assets or systems, but it focuses more on the process as a whole rather than on the operation of a specific piece of equipment.
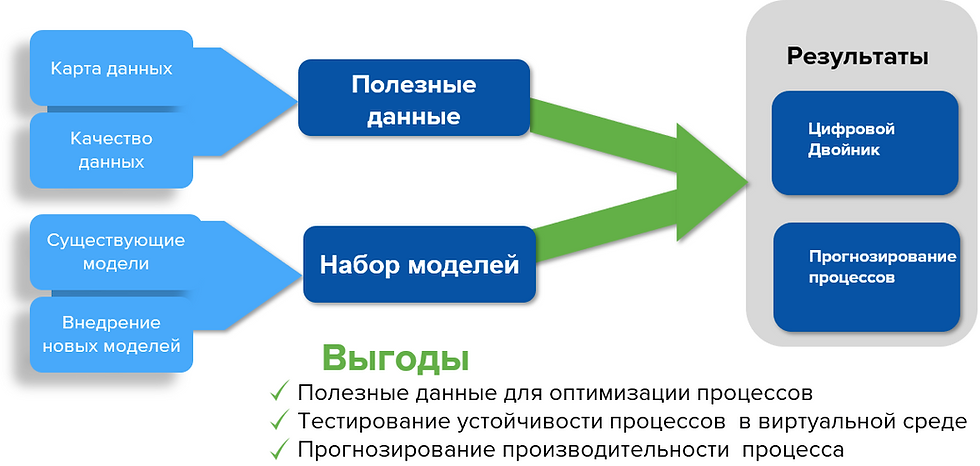
What tasks can digital twin technology solve?
Since the digital twin has all the information about the equipment, the company has new opportunities:
1. By using a digital twin, it becomes possible to concentrate the bulk of changes and costs at the design stage , which reduces costs that arise at other stages of the life cycle .
2. Know about malfunctions in a timely manner. Data from sensors is updated in real time. And technicians always see whether the equipment is working properly or whether an anomaly has occurred that could lead to a failure or accident at work.
3. Predict equipment breakdowns and wear. Using the data obtained from the digital twin, it is possible to plan maintenance and replace parts that are likely to fail in advance.
It is very important not to confuse the types of forecasting. Recently, working with the market of various IT solutions, a trend has been noticeable of mixing the concepts of predictive analytics and machine detection of deviations in the operation of equipment.
That is, using machine detection of deviations, we are talking about the introduction of a new, predictive approach to organizing service.
And, on the one hand, neural networks really work in both places. In machine detection of anomalies, neural networks also detect deviations and it is possible to organize maintenance before a serious breakdown and replace only the worn-out element.
But let's take a closer look at the definition of predictive analytics.
Predictive (or predictive, forecasting) analytics is forecasting based on historical data.
And in terms of working with reliability, for us this is an opportunity to predict equipment failures before deviations occur. When operational indicators are still normal, but tendencies towards deviations are already beginning to form.
If we translate it to a very everyday level, then the detection of anomalies is when your blood pressure starts to change and you are warned about it, before your head starts to hurt or you start having heart problems.
And predictive analytics is when everything is still normal, but your diet, quality of sleep, or something else has already changed, and processes begin to occur in the body that will lead to an increase in pressure.
And the main difference is the depth of immersion, the presence of competencies and the prediction horizon. Identifying anomalies is a short-term prediction, so as not to bring it to a critical situation, it is not necessary to study historical data here.
And full-fledged predictive analytics is a long-term forecast. You have more time to make a decision and develop measures. Either plan the purchase of new equipment, spare parts and call a repair team at a lower price, or take measures to change the operating modes of the equipment and prevent deviations from occurring.
4. Optimize production operations. Over time, the digital twin accumulates data on the operation of the equipment. By analyzing it, you can optimize the operation of the enterprise and reduce costs.

Examples from life:
Tecnomatix created a digital twin of production for PROLIM, which wanted to improve the product assembly process. To begin with, a visual twin of production was created. Then, data on the speed of movement of objects, the number of workers and their performance, and many others were taken from sensors. All this information made it possible to create a digital twin that repeats all the processes of a real object.
Tesla creates a digital twin for every car it sells. Its built-in sensors transmit data to the factory, where artificial intelligence decides whether the car is working properly or needs maintenance. The company even fixes some glitches remotely by simply updating the car’s software.
Chevron Corporation is using the technology in oil fields and refineries to predict potential technical problems. The use of digital twins in manufacturing is expected to expand by 2024.
Singapore has a digital twin — a dynamic 3D model of the city with all its objects: from buildings and bridges to curbs and trees. The virtual Singapore receives data from city sensors and information from government agencies. The positive effects of implementing a digital twin of the city: the government can predict actions in case of an emergency, and architects can plan new construction projects taking into account the city's infrastructure.
The main limitation at the moment, I see, is the complexity and high cost of the technology. It takes a long time and is expensive to create these models, the risk of errors is high. It is necessary to combine technical knowledge about the object, practical experience, knowledge in modeling and visualization, compliance with standards in real objects. This is not justified for all technical solutions, and not every company has all the competencies
For now, this is a new technology. And according to the same Gartner cycle, it must pass through the "valley of disappointment." And later, when digital competencies become more commonplace and neural networks become more widespread, we will begin to use digital twins to the fullest extent.
The concept of digital transformation implies the active use of cloud technologies, online analytics and remote management capabilities.
The National Institute of Standards and Technology of the United States has identified the following characteristics that characterize clouds:
Self-service on demand - the consumer independently determines his computing needs: server time, access and data processing speeds, volume of stored data - without interaction with a representative of the service provider;
Universal network access - services are available to consumers over the network, regardless of the device used for access;
Resource pooling - a service provider combines resources to serve a large number of consumers into a single pool/pool for rapid redistribution of capacity between consumers when demand for capacity changes; in this case, consumers manage only the basic parameters of the service (e.g., data volume, access speed), but the actual distribution of resources provided to the consumer is carried out by the provider (in some cases, consumers can still manage some physical parameters of redistribution, for example, specifying a desired data processing center based on geographic proximity);
Elasticity - services can be provided, expanded, or reduced at any time, without additional costs for interaction with the supplier, usually in automatic mode;
Consumption accounting - the service provider automatically calculates the consumed resources at a certain level of abstraction (e.g. the volume of stored data, bandwidth, number of users, number of transactions) and, based on this data, estimates the volume of services provided to consumers.
Now let's consider why we should implement clouds? What advantages does it provide?
This approach allows:
do not "freeze" finances by investing in fixed assets and future expenses (for repairs, renovations and modernization). This simplifies accounting and tax management, and allows resources to be directed toward development;
save on payroll (salaries + taxes for expensive specialists to service the infrastructure) and operational costs (electricity, rent, etc.);
save time on startup and initial use;
more efficient use of computing power. No need to build a "redundant" network to cover peak loads, or "suffer" from system "slowdowns" and risk a "fall" with data loss. This is the "provider's" task, and he will do it better. Plus, the principle of division of responsibility is included, and data safety is his task.
availability of information both in the office, at home and on business trips. This allows us to work better and more flexibly, and hire people from other regions.


There are many models for using cloud technologies: SaaS, IaaS, PaaS, CaaS, DRaaS, BaaS, DBaaS, MaaS, DaaS, STaaS, NaaS
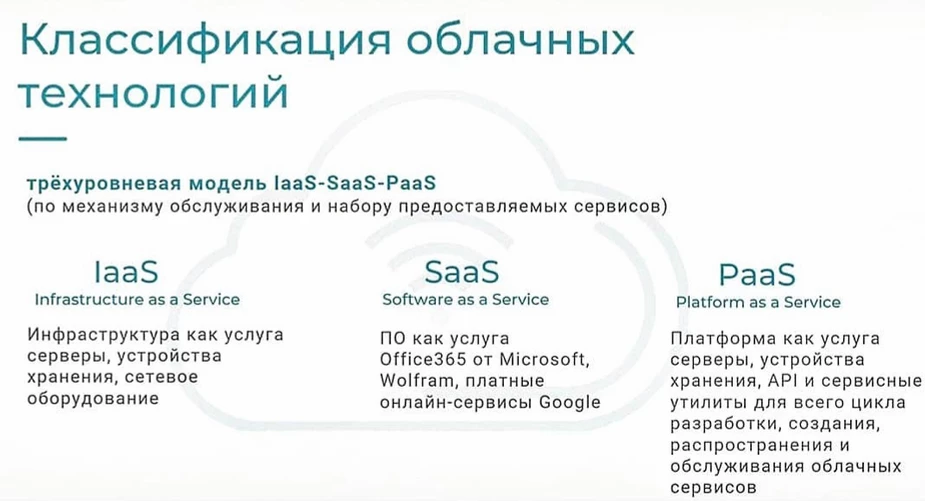
SaaS (Software as a Service) - software as a service
The most widespread in the world, used by almost all people with Internet access.
The client receives software products via the Internet. For example, mail services mail.ru. Gmail, or the cloud version of 1C.
IaaS (Infrastructure as a Service)
Providing computing resources for rent in the form of virtual infrastructure: servers, data storage systems, virtual switches and routers. Such IT infrastructure is a full-fledged copy of the physical one.
PaaS (Platform as a Service)
Rent a full-fledged virtual platform, including various tools and services. The client can customize such a platform to their needs, making it a platform for testing software or, for example, a system for automating the control system. The service is especially popular with software developers
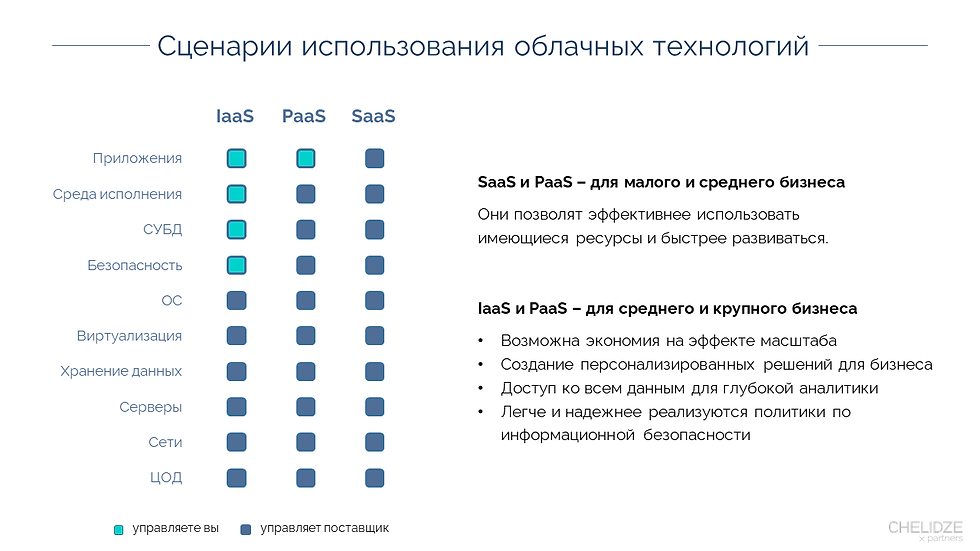

CaaS (Communications as a Service)
This service consists of providing clients with various communication tools in the cloud. This could be telephony, instant messaging services or video communication. All the necessary software is located in the provider's cloud.
WaaS (Workplace as a Service)
In this case, the company uses cloud computing to organize the workstations of its employees, setting up and installing all the necessary software required for the staff to work.
DRaaS (Disaster Recovery as a Service)
This service allows you to build disaster-resistant solutions using the provider's cloud. The cloud service provider's site is a "spare airfield" to which data from the client's main site is constantly replicated. If the client's services fail, they are restarted within a few minutes, but in the cloud. Such solutions are especially interesting for companies with a large number of business-critical applications.
BaaS (Backup as a Service)
This type of service involves ensuring the backup of the client's data to the provider's cloud. The cloud service provider provides the customer not only with a place to store backup copies, but also with tools that ensure fast and reliable copying. For the correct implementation of this service, the planning stage is very important, during which the parameters and depth of the archive, as well as the bandwidth of data transmission channels, must be calculated.
DBaaS (Data Base as a Service)
This cloud service consists of providing the ability to connect to databases deployed in the cloud. The client pays for rent, depending on the number of users and the size of the database itself. It is worth noting that such a database will never fall due to lack of free disk space.
MaaS (Monitoring as a Service)
This type of cloud service helps to organize monitoring of IT infrastructure using tools located in the provider's cloud. This is especially important for companies whose infrastructure is geographically dispersed. This service allows you to organize centralized monitoring of all systems with a single entry point.
DaaS (Desktop as a Service)
This service consists of providing users with remote desktops. With this service, you can quickly and cost-effectively organize a new office with centralized management of workstations. Also, one of the advantages of this service is the ability to work from any device, which is especially valuable for employees on business trips and constant travel.
STaaS (Storage as a Service)
This service consists of providing disk space in the provider's cloud. At the same time, for users, such space will be a regular network folder or local disk. The strong point of this solution is increased data security, since reliable data storage systems operate in the provider's cloud.
NaaS (Network as a Service)
This service allows you to organize a full-fledged, complex network infrastructure in the provider's cloud. This service includes routing tools, security organization, and the use of various network protocols. To visualize how this works, I want to give an example from one TV series - Zhuki.
The main problem in it throughout the 2nd season is that the purchased equipment for the mobile application cannot work stably: either there is not enough power and the equipment heats up, or the power of the power grid. When one of the servers fails, the entire system crashes. And one of the partners before that copies the entire database and tries to escape with it.
As we can see, the system has many limitations and bottlenecks.
If the characters in the series had chosen IAAS or PAAS, they would have:
1. At the start, you wouldn't have to worry about losing a bag of money. You wouldn't have to buy the cheapest, least productive, and most unreliable equipment.
2. You wouldn't have to think about cooling and overheating. You can work calmly from your laptop in comfortable conditions.
3. There would be no need to arrange a quest to steal a transformer from a neighboring village.
4. There would be no scenes of jealousy and the entire service would not be down due to a server failure.
5. The partner would not be able to copy the entire database to an external drive and erase everything from the laptop.
Another criterion by which clouds should be divided is the deployment model:
A private cloud is an infrastructure designed for use by a single organization that includes multiple consumers (e.g., departments of a single organization), and possibly also customers and contractors of that organization. A private cloud may be owned, managed, and operated by the organization itself or by a third party (or any combination of the two), and may physically exist both within and outside the owner's jurisdiction.
A public cloud is an infrastructure intended for free use by the general public. A public cloud may be owned, managed, and operated by commercial, academic, or government organizations (or any combination thereof). A public cloud physically exists in the jurisdiction of the owner—the service provider.
A community cloud is a type of infrastructure intended for use by a specific community of consumers from organizations that share common goals (e.g., mission, security, policy, and compliance requirements). A community cloud may be cooperatively owned, managed, and operated by one or more community organizations or a third party (or some combination of the two), and may physically exist both within and outside the owner's jurisdiction.
A hybrid cloud is a combination of two or more different cloud infrastructures (private, public, or community) that remain unique entities but are linked by standardized or proprietary data and application technologies (e.g., short-term use of public cloud resources to balance loads between clouds).
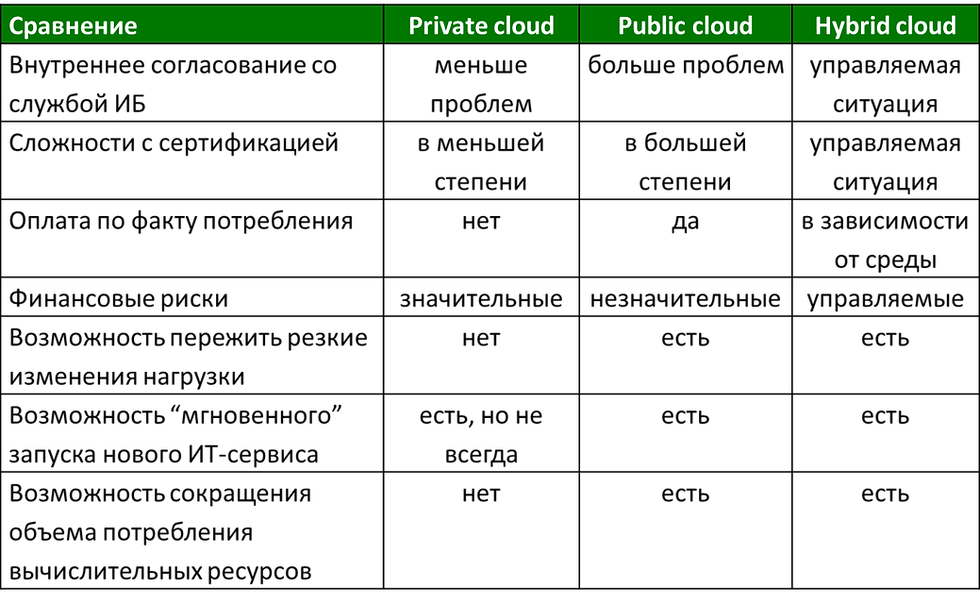
Disadvantages of cloud computing:
Demanding Internet reliability. Sometimes a constant and reliable connection is required.
Working with data. Not all SaaS solutions are willing to share your data. When choosing a supplier, consider its approach in this direction: are there convenient tools for uploading data to their storage, are they ready to share it with you at all. After all, you are on the way to using end-to-end analytics and data science. And data is the blood for this.
Internet speed requirements. Some applications do not work well with slow Internet.
Performance limitations may apply. Programs may run slower than on the local computer.
Not all programs or their functionality are available remotely. If we compare programs for local use and their "cloud" counterparts, the latter are still inferior in functionality.
High cost of equipment – to build your own cloud. The company needs to allocate significant material resources, which is not beneficial for newly created and small companies.
The confidentiality of data stored on public clouds is currently a matter of much debate, but in most cases experts agree that it is not recommended to store the most valuable documents for a company on a public cloud, since there is currently no technology that would guarantee 100% confidentiality of stored data.
Data security may be at risk. This creates restrictions in the information security policies of large companies. But the key word here is "may". It all depends on who provides "cloud" services. If this someone reliably encrypts data, constantly makes backup copies, has been working in the market of such services for many years and has a good reputation, then a threat to data security may never happen. If data in the "cloud" is lost, then it is lost forever. This is a fact. But it is much more difficult to lose data in the "cloud" than on a local computer.
Well, in conclusion, I want to share an interesting infographic that will help you decide whether it is necessary?
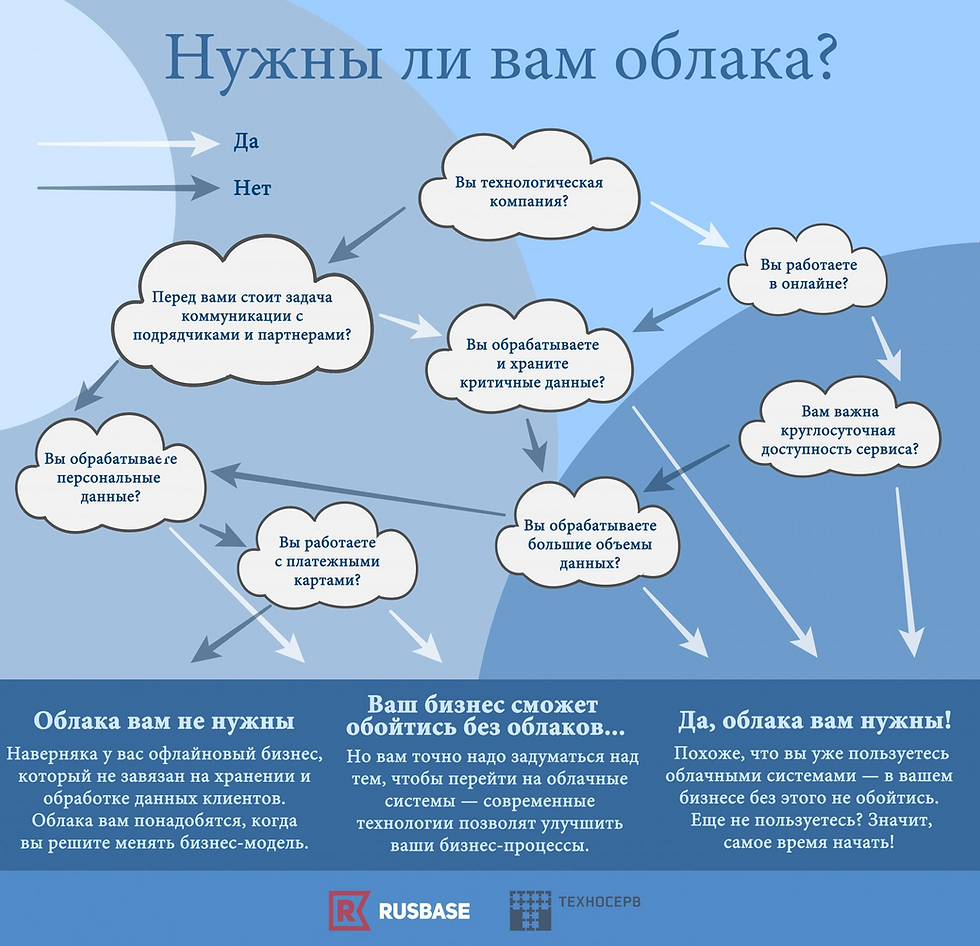
But my private and personal opinion is that clouds are needed even for offline businesses. As I wrote above, it helps to organize remote work for managers (to be productive everywhere) and hire the best personnel, regardless of the territory.
Digital platforms are one of the foundations of the transition to a digital economy. This is a reduction in the costs of processing information not only on the scale of one process and company, this is a radical restructuring of the market. Thanks to this, services and goods become more accessible, better quality and cheaper.
A platform is a product or service that brings together two groups of users in two-sided markets.
Two-sided markets are network markets that have two sets of users with network effects occurring between them. A two-sided network has two categories of users whose purposes for using the network and their roles in the network are clearly distinct.
In scientific terms, a digital platform is a system of algorithmic relationships between a significant number of market participants, united by a single information environment, leading to a reduction in transaction costs through the use of a package of digital technologies and changes in the division of labor system.
An example from our, Russian, life - Yandex Taxi. Yandex does not own anything except the program and the platform, and connects you with other partners (self-employed, taxi companies, etc.). Yandex is an aggregator and gives you a "single" window for communicating with everyone and receiving a service, taking an average of 20-25% of the order cost from drivers for this.
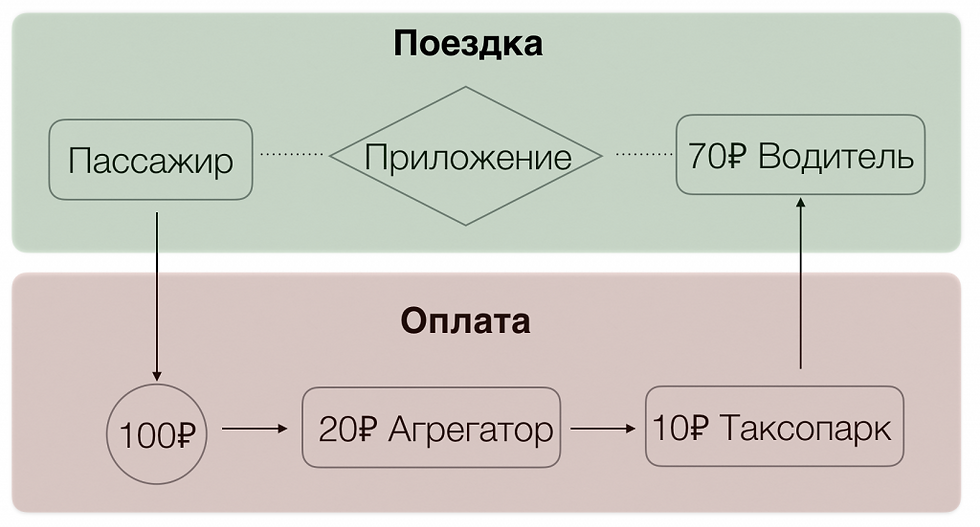
The main criterion for any platform is a large number of participants so that a "network" market can emerge.
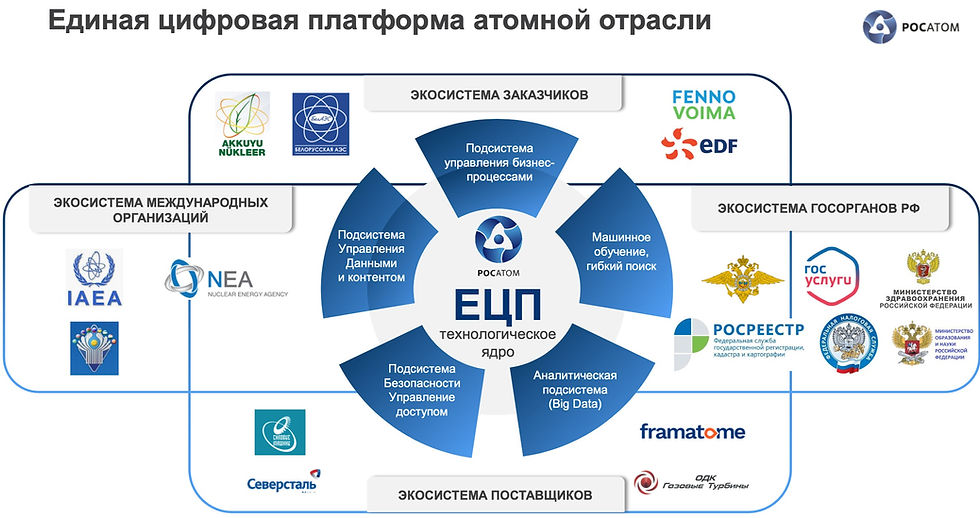
But let's look at an even more global scale - Aliexpress.
Almost every one of us is familiar with this service. But what is Aliexpress?
This is a small platform in the Alibaba ecosystem (according to various sources, it is in the TOP-10 world companies with a capitalization of about 620 billion dollars ). Below is a diagram with the interrelations within this ecosystem.
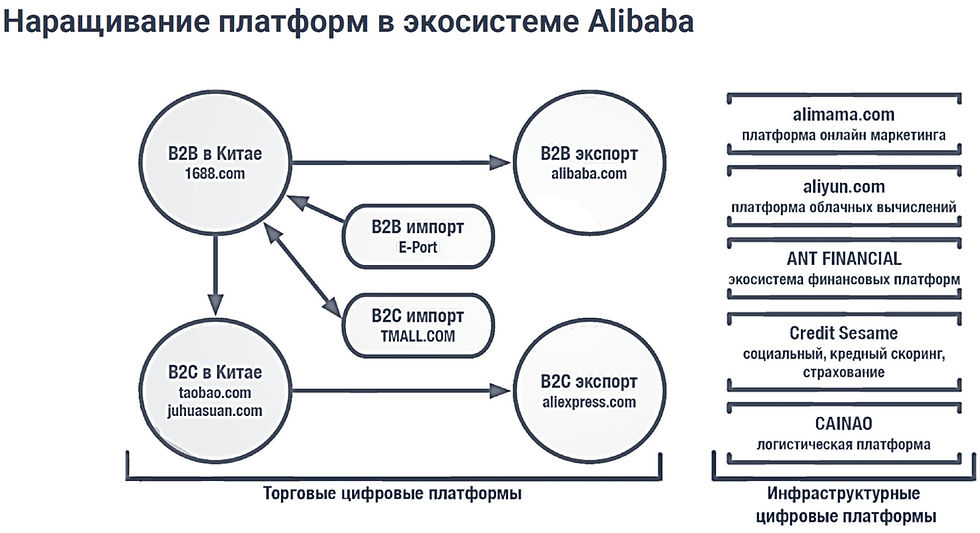
And many entrepreneurs in our country work based on interaction with these platforms. They order, for example, on taobao and deliver.
So how does the price decrease for us? Below is a visual diagram.

Platforms also come in different types and classes. Below is the classification proposed by RANEPA.
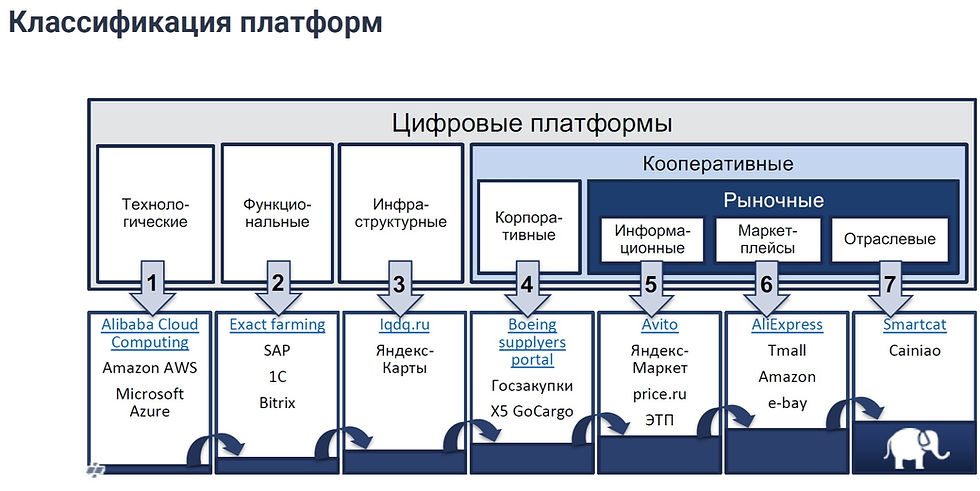
The emergence of platforms is a radical restructuring of markets. We looked at Yandex Taxi above. Due to their advantages, they changed the entire taxi market in the country. Many taxi companies have already stopped working with their own orders. They connect to one of the aggregators - Yandex, Gett, CityMobil. And now their main source of income is renting cars to drivers.
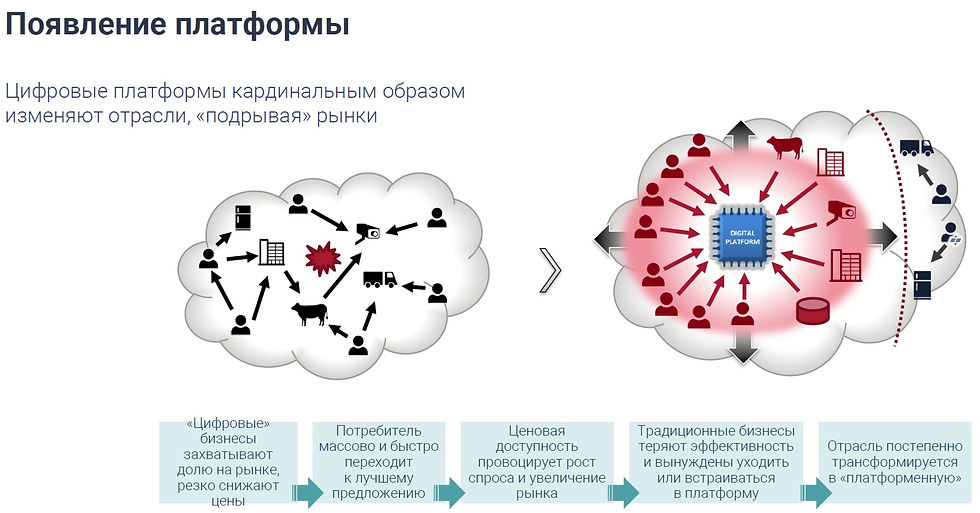
Also, it was said earlier that services and goods are becoming better. But how?
Open platforms allow working with ratings and reviews. And users can choose the most reliable partners.
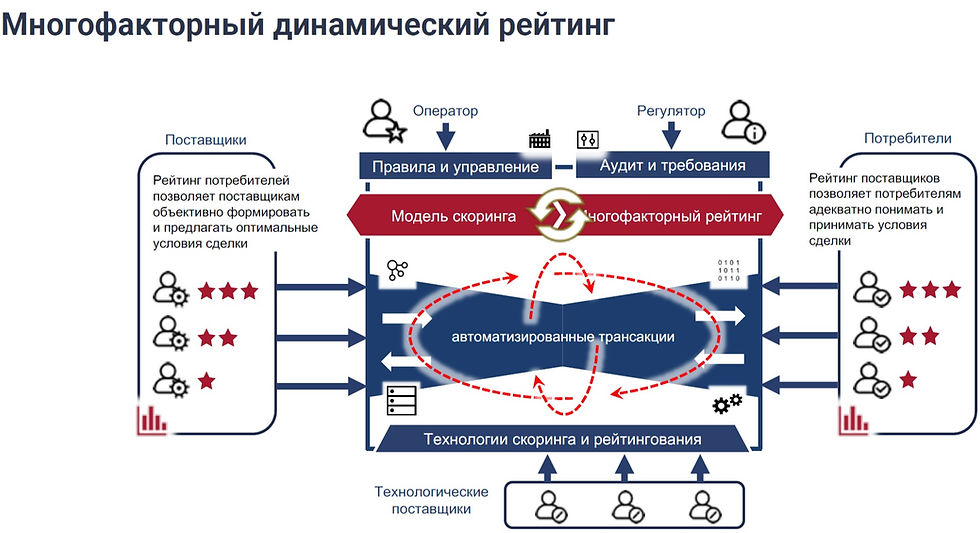
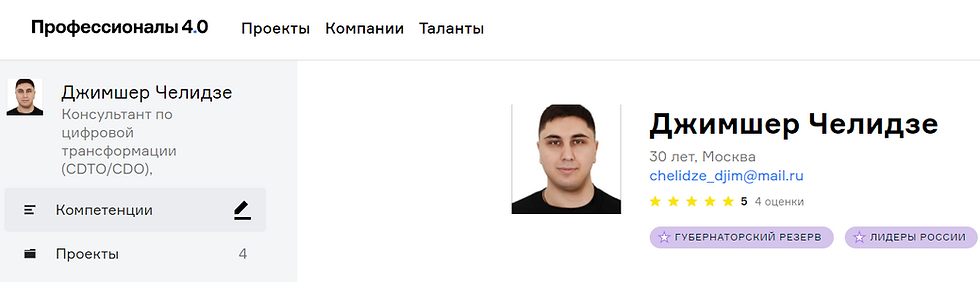
In addition, platforms allow for market “development”: collecting consumer needs and quickly assessing the viability of new product/service ideas.
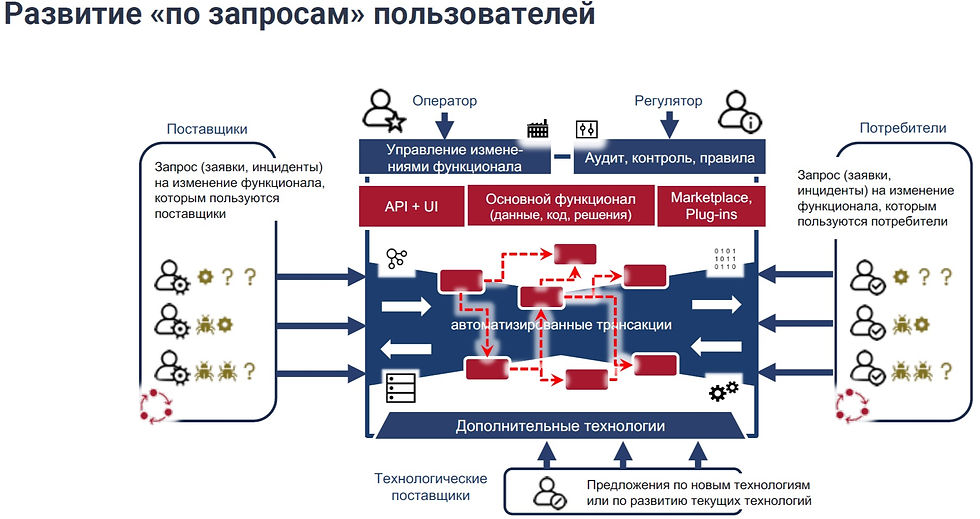
Limitations and risks
Complexity of regulation.
Currently, many popular platforms are not regulated in any way, which creates conditions for abuse by their operators. Especially, given the dominant or monopolistic position of the operators of these platforms. Small service and product providers suffer from this especially. They already have a large consumer base, and they take advantage of it.
Unfair competition.
Platform operators have all the data and analytics: what exactly is being sold, in what quantities, what is the description of these goods, their properties, who is the most "resourceful" and suppliers. As a result, it becomes possible to use black PR and unfair competition.
The difficulty of working on some of them, lack of competence.
Not all "customers" and "suppliers" have all the competencies to work on such platforms. As a result, stories of millions in losses are not uncommon.
Key findings from the study* on the reasons for platform company failures:
Because many things can go wrong in the platform market, managers and entrepreneurs must coordinate their efforts and learn from failures. Despite the huge growth opportunities, a company’s platform strategy does not necessarily increase the chances of business success.
Since platforms ultimately thrive on network effects, the key challenges remain getting prices right and choosing the right side to subsidize. Uber has been great at calculating (and Sidecar has been fatally missing) the power of network effects on transaction volume, with prices and costs plummeting for both sides of the market. While Uber is still struggling with the economics of the project (and may yet fail as a business), Google, Facebook , eBay, Amazon, Alibaba, Tencent, and many other platforms have started by aggressively subsidizing at least one side of the market and have become highly profitable.
Trust should be the first concern. Asking customers or suppliers to act at their own risk without any history of dealing with the other party is too much for any platform. EBay failed to create trust-building mechanisms in China, but Alibaba and Taobao succeeded. Platform leaders can and should avoid such mistakes.
Timing is critical. Being first is preferable, but it is no guarantee of success: remember Sidecar. Being late, however, can be deadly. Microsoft’s disastrous delay in creating a competitor for iOS and Android is a case in point.
Finally, arrogance is also a recipe for failure. Even if you have an unconditional advantage, ignoring the competition is unforgivable. If you cannot remain competitive, no market position is safe. Microsoft's terrible implementation of Internet Explorer is a case in point.
* David Yoffie is a lecturer in the Department of International Business Management at Harvard Business School; Annabel Gawer is a senior lecturer in the digital economy and director of the Centre for the Digital Economy at the University of Surrey, UK; Michael Cusumano is an emeritus professor at the MIT Sloan School of Management, Cambridge.
Augmented reality (AR) is a technology that complements the real world by adding any sensory data.
A visual example of this technology in action can be seen in the 2 diagrams from Harvard Business Review below.
Mixed reality ( MR ) is a further development of AR, where objects of the virtual world interact with objects of the real world to a limited extent.
Virtual reality (VR) is a virtual world created with the help of hardware and software, transmitted to a person through touch, hearing, as well as sight and, in some cases, smell.
Resume:
AR – is a real environment with virtual digital objects;
MR – a real environment with virtual objects that you can interact with;
VR is a completely virtual environment.
We are most familiar with these technologies from various games, such as Pokemon Go, 3D-5D cinemas, and so on.
But as with 5G, the potential of this technology is much broader than the usual "hobbyist" use and entertainment.
Several main directions of development of the industry can be identified:
games;
movie;
sports broadcasts and shows;
social media;
marketing
education;
medicine;
trade and real estate;
industry and military-industrial complex.
Some real examples:
An AR program created by Microsoft and Volvo shows the structure of a car's engine and chassis.
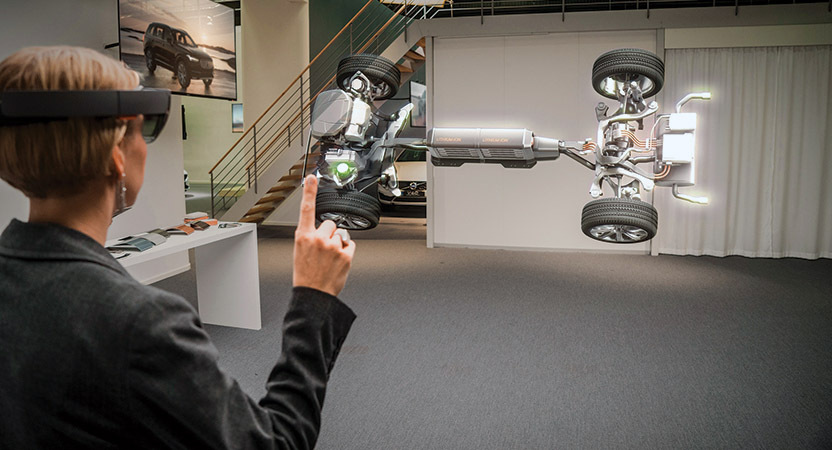
Porsche has implemented augmented reality technology to organize the maintenance of its cars.
Ford is using VR to host workshops where engineers from different locations around the world work with holograms of prototype cars in real time. They can walk around life-size 3D holograms and even “climb” inside to assess the layout of the steering wheel, controls, and instrument cluster. No more expensive physical prototypes and no more gathering engineers in the same workshop
The benefits provided by technology:
Manufacturing. In the industrial sector, augmented reality can increase productivity by reducing the human factor and provide a competitive advantage by increasing work efficiency.
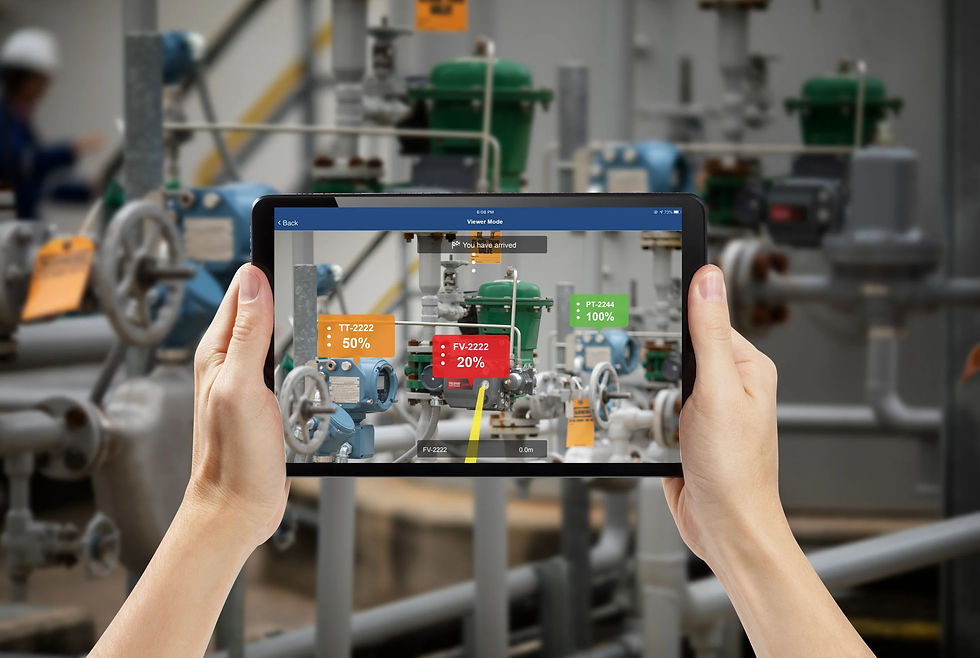
Retail. Millions of people in our world make online purchases, which allows them to save time and money. But the choice of goods does not always coincide with the desired result. AR applications make it possible to clearly see the product purchased online and avoid problems with the correct choice.
Navigation. Using navigation applications allows you to better navigate in space, indicating clear directions to the desired objects.
Maintenance and repair industry. AR applications enable people without professional skills to perform minor repairs when there is no appropriate service center nearby. This is clearly seen in the example of Inglobe Technologies for car maintenance.
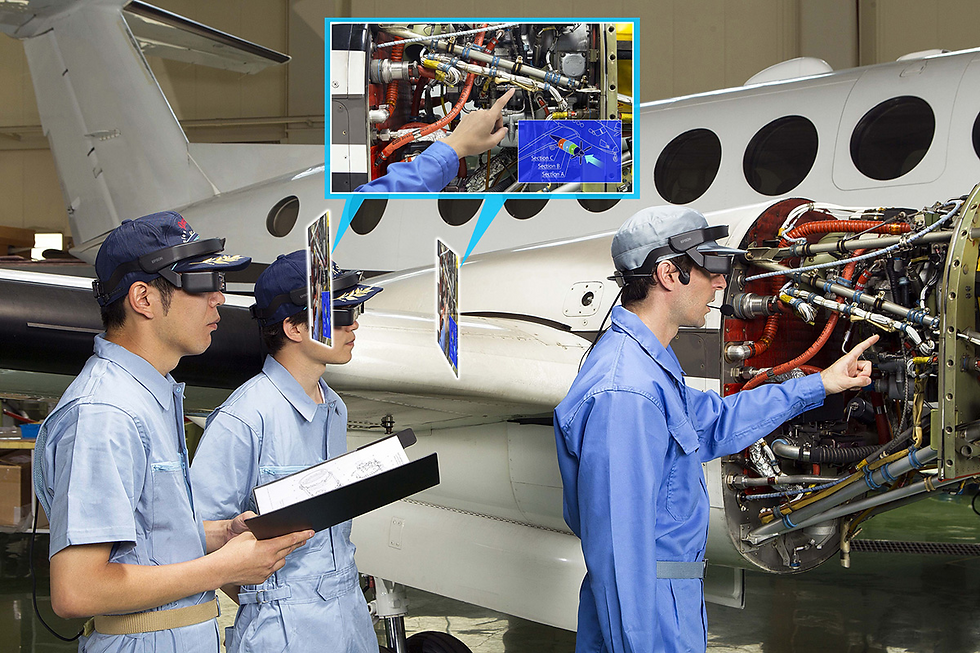
Limitations and disadvantages:
There are few qualified specialists to create models. But this problem is being solved. And soon there will be quite simple platforms, like website builders.
Demanding hardware performance. This problem has also been practically solved.
Lack of trust among people and investment funds.
My personal opinion is that this is also one of the most promising technologies, and the companies that work on it have huge growth potential.
We are developing our own digital solution for your projects. You can find it here: